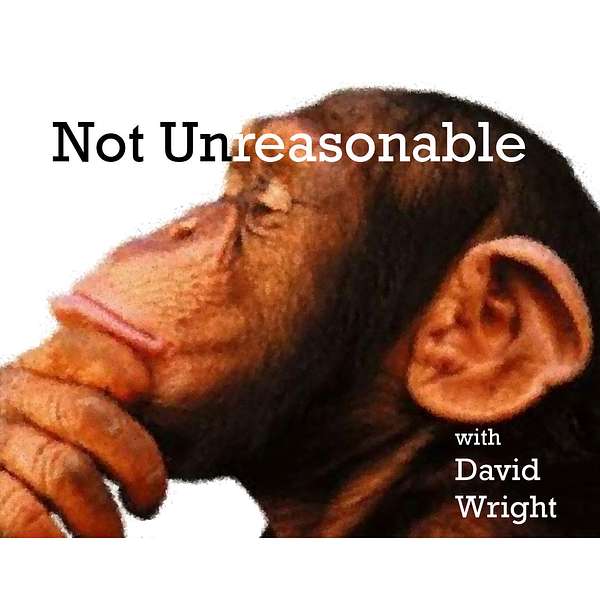
The Not Unreasonable Podcast
Subscribe in iTunes, stitcher, or by rss feed. Sign up for my newsletter here and also see us on youtube!
Show notes at notunreasonable.com
The Not Unreasonable Podcast
Pricing Insurance Risk With Steve Mildenhall and John Major
Nobody knew how to price volatility until now. I bet you're surprised!
This isn't hyperbole, Steve Mildenhall and John Major have a deep and thorough understanding of all the relevant literatures and have been part of a loosely collaborative team of academics and actuaries working out the details of a coherent, actionable theoretical foundation for pricing insurance for their entire careers. Now that they're both retired they've delivered us the tome the actuarial profession needs.
Their new book: *Pricing Insurance Risk: Theory and Practice* is an absolute masterpiece. It is theoretically sound and immensely practical. Until today no financial institution could choose a sophisticated portfolio model that wasn't hiding biases or inefficiencies that had to be 'band-aid-ed' over with coarse heuristics. Very importantly how does one calculate the margin required to service the capital base when the risks vary so much? The science of managing a portfolio of incompletely diversified, highly volatile financial instruments has gained serious ground with this book.
Steve Mildenhall is head of analytics at Qualrisk, an Insurance consulting firm, formerly assistant professor of actuarial science at St. John's University, and before that CEO of analytics at Aon. This is Steve's fifth appearance on this show. John Major is principal at Major analytics and the former director of actuarial research at guy carpenter.
youtube: https://youtu.be/ZQHpMVH7d9s
show notes: https://notunreasonable.com/?p=7694
Steve and John did a tutorial on this material and more with some technical examples you can see here: https://www.youtube.com/@ermdiner/
Here is a link to the spreadsheet used in the tutorial:
https://docs.google.com/spreadsheets/d/1CV3sF52cjPH8mw6T4E-jOfCNDagCptRs4xBZTug8Z0A/edit?usp=sharing
Here are some Not Unreasonable Podcast Episodes about related content:
Samir Shah on Innovating Capital
https://www.buzzsprout.com/126848/8171216
and
Steve on the Macro History of insurance Part 1:
https://www.buzzsprout.com/126848/8121657
And Part 2
https://www.buzzsprout.com/126848/8507268
In the show we cover:
How do you think of the cost of running the capital side of insurance?
2:06
The importance of connecting the value to the value of the original customers.
5:18
What is the value of reinsurance?
7:34
The timing of Hurricane Andrew relative to the early cat models.
18:44
The history of cat models in the 60s.
21:22
What the CEO’s are disagreeing about in volatility?
33:04
What could possibly justify 50% margins in these companies?
42:59
What doesn’t make sense about the intentionality argument.
46:28
What is the market price for risk?
48:02
The difference between allocating capital vs. allocating margin.
1:01:39
What’s the right metric for determining performance of a reinsurance company?
1:08:07
The further removed you get from loss, the cheaper the capital is as a percent of capital, but the more expensive the insurances are.
1:22:59
How long did it take for these ideas to emerge? How did they evolve?
1:24:55
The key to unlocking a lower cost of capital
1:29:07
What is the required return in a regulated environment?
1:32:55
The amount of leverage you get is huge.
1:48:24
Steve’s envelope theorem and how it works.
1:53:18
What’s the insight that underlies the ability to determine bounds for spectral measures?
1:56:46
Characterizing the worst risk-adjusted expected outcome.
Twitter: @davecwright
Surprise, It's Insurance mailing list
Linkedin
Social Science of Insurance Essays
My guests today are Steve Mildenhall and John Major. Steve is head of analytics at Qualrisk, an Insurance consulting firm, formerly assistant professor of actuarial science at St. John's University, and before that CEO of analytics at Aon. This is Steve's fifth appearance on this show. John is principal at Major Analytics and the former director of actuarial research at Guy Carpenter. And this is his first time on this podcast, Steve and John have written a magisterial new book called Pricing Insurance Risk: Theory and Practice, which we'll be using to trace the past, present and future of that topic today. And I'm very excited to have you on the show. Welcome.
John Major:Great to be here.
David Wright:First question. So let's say you're wildly successful with this book. And actuaries master this material. How will insurance customers be better off at the end of that process than they were when you both started your careers? Let's say kind of in around 1990.
John Major:Well I don't know if 1990 is the right anchor point for that. You know, this was before Hurricane Andrew before the Northridge earthquake. And I think it's fair to say that back then catastrophe risk was severely underpriced. So customers were doing pretty well back then. Yeah, you want to go forward to something like 2010. And now you're in a situation where it's, you know, you have cat modeling, the stuff came out of the laboratory, and it's commercialized, and you have a pretty good consensus for, for better or worse as to what cat risk looks like. You can at least take a view of it. There's uncertainties involved, of course, but you can take a view on on your loss cost, and then seriously address the question of the risk load, the risk margin, and that's what our book is about. It's about
David Wright:You might say, though, that the customers thought they were better off, but they weren't, right. I mean, we're in the world of unusual things and insurance. And so the customers all thought they're getting a pretty good deal. But the ones that got their insurance companies went insolvent after Andrew probably realized after the fact that they weren't. Steve, what do you think?
Steve Mildenhall:Yeah, so I was I was gonna begin by saying that I hope, before we get to the customers, actuaries will be better off because I think one of our motivations in writing this book was that the current exam syllabus and education, the preparation we give the new fellows and Associates is not great in this area. I mean, there's some really good papers on the syllabus, but that very much from the sort of developmental phase rather than actually the clean, crisp presentation of an established theory. So partly, we just hope that actuaries have a more productive time. And so the cleaner set of conversations when discussing these topics with, with management, their employees, in terms of customers, I guess, I look at it maybe sort of step back a bit, right, I think that the idea of managing risk through an insurance pool, so pooling risk, taking the advantage of diversification, is kind of truly one of the five great ideas of mankind, right? I might have put it right up there with the wheel and fire em and money and money, right? Division of labor, that type of thing. And in order for that to function, you have to bring together a group of people who are strangers to one another. And so it's really important that the pool treats the, the members fairly, it needs to, it needs to be seen to treat them fairly. And it needs to sort of demonstrably treat them fairly, right. And if you look at a lot of the infrastructure, we have set up around insurance, regulation, rating agencies, and so forth. Let's make sure we actually back our promises up, we pay our claims. On the loss cost side, there's a lot of work around your disparate effects, unfair discrimination on that type of thing. What we're filling in with this book is the profit component piece of that, right, we are this book is really about how do you think of fairly allocating the cost of running the capital side of your insurance? And so yeah, I would see this, this work as part of the sort of ongoing efforts to ensure that the risk bearing function of insurance pools operate as effectively as possible. And as John is brought out, it really is all about the capital, right? I mean, that is, you know, the margins on both lines of businesses, you know, maybe sort of five to 10% of the expected loss. Whereas the margins on cat business by you know, earthquakes, hurricanes, floods, the really big events, it's a multiple of these. So there's two or three 500% of expected loss. So it's really, really important. And it tends to, if that part of the market fails the whole thing gums up as we saw after Andrew as we saw after Northridge as we saw after 2006 Yeah, I'm hearing that this this year end renewal is going to be pretty, pretty interesting as well. So I... I think the way customers are going to benefit is not necessarily they're gonna get what they regard as cheaper prices, but they're gonna get fairer prices, and they're gonna be able to buy insurance in a market that operates as effectively as possible and provides as broader set of coverages to them as possible.
David Wright:So I'm very happy. I like, I like that answer, Steve. And I think that in the first part, I was a little worried there, because I think you'd be doing yourself both a disservice by not connecting it to what the because let's face it, actuaries do serve customers, in a abstracted kind of sense or distant sense. There's a few layers of people in between actuaries and customers. So I might say that's a good idea. But there is a purpose is a customer serving purpose to the actuaries work. And I think a lot of ways of this phenomenal book, phenomenal book, which is definitely a book for actuaries. So not every listener to this podcast, will want to buy this book, but they can all benefit from the concepts that we're gonna be discussing today. And I think that actuaries often have there it is a, probably a, sometimes a hard time connecting the work they do to the value to the original customers. I think you did a really good job there, Steve, of talking about how they, how the concept of a stable insurance industry is very much what you guys are supporting and advancing with this work. But I have a question then, which sort of follows on from this concept? How do you describe to people what you do if somebody off the street, right, friend of a friend shows up? Oh, hey, what do you do for a living? What do you say?
Steve Mildenhall:I say I'm retired.
David Wright:Okay, what did you say? 10 years ago? Steve, you can't get away with that. Not today.
Steve Mildenhall:It's about so every one will say risk return right? You hear you go and look at your mutual funds. And you're deciding where to invest. You hear this expression risk return all over the place, high risk, high return? Well, what this book is about is how much quantified how do you quantify and measure the risk, which is a really slippery character, right? Risk is a very nuanced, multi dimensional thing. It's hard to pin it down to one number, how do you characterize the risk? And then you can measure the return. That's generally easier, not necessarily always completely easy. But how are you relating the return to however you've decided to measure the risk? So it's all about for the quantifying? What does it mean, when I say high risk means, ie, you know, requires a higher return?
John Major:Yeah, the the question of risk versus value
David Wright:John? has been something I've been chasing for years and years and years. I was working for a reinsurance broker in the last part of my career. And of course, we're trying to make deals for reinsurance. And so the natural question is, well, what is the value of reinsurance? And, you know what? Nobody had a really good answer to that. And it's amazing that they didn't. And it was also, it's one of the situations where problems worthy of attack prove their worth by fighting back. A lot of really brilliant people, over the years have been trying to tackle this question, with varying degrees of success. And so I think what we did in this book here is kind of filtered of a heck of a lot of really good research done by a lot of people and distill down the parts that worked, and saw and showcase how they all fit together into this answer. It's not like we did a lot of original research. In this particular book. I mean, we did, we did our own individual research in the years past, contributing our, our little streams to the rivers of research. But, but in the book, we pull together this incredible body of research that had been pretty opaque to the people who need to understand it to the actuaries to the working actuaries.
Steve Mildenhall:Yeah, I mean, I add to that, so I always felt like when I was working, there's a lot of economists and finance guys, you know, write papers and what have you. And in insurance is such an obvious thing to for them to study, this is sort of pure risk. There's got to be a good answer to this out there. It's just a matter of finding and understanding. And, you know, if I had to, like identify one paper that that I think has sort of the most interesting and the most important answers that we, you know, explore in the book. It was written in the year 2000. And I probably first started looking at it and reading it and trying to understand it in you know, fairly soon thereafter. And I remember, you know, I would keep going back to it over and over again, because it was a fairly, you know, math paper basically, it wasn't, it wasn't a very, you know, easy to read paper and Sure enough, it turns out when I finally had the time to dig through and understand what it was saying, it has the answers and it has, you know, as the answer to the age old problem of, you know, when you do a marginal calculation, you have what's described as ordering problem, right? Do I think of, it seems like the allocation of costs you get depends on what order you bring your risks into the portfolio, which is obviously a bit of a problem. And it turns out, the reason for that is you're trying to differentiate, when you do marginal, you're really talking about slopes, right? You're trying to differentiate a function that's got a cusp. So it matters, whether you approach it from the left or the right, you get a different slope, it was right there in this paper. And it not only you know, identifies that identifies that if that isn't the case, you don't have that problem. And it gives sort of a really nice of a wrap up answer to, to unifying two ways of looking at risk
measure:a marginal way, and a risk adjusted probability way that sort of seem to be different in a lot of cases, but actually are the same, they give you the same answer, provided you have the right underlying assumptions. And that was just a joyous discovery. It wasn't a discovery, it was joy learning out of out of the literature once I finally understood what it was saying.
David Wright:So I am going to
John Major:Let me amplify it, let me amplify on it. So it is in a nutshell, there's the answer sitting out there a mid 1000 other papers, a mid hundreds of other papers that taking ridiculous, you know, continuous time option pricing models, approach to insurance pricing, and and there's the answer out there. And it takes Steve Mildenhall, above average intelligence among the actuaries, 20 years to figure it out what's really going on here. So it's like, it's like panning for gold. You know, you know, it's out there. But you know, it takes a lot of time to find it.
David Wright:Yeah, I mean, so... a few thoughts. One, we're gonna come to Freddy Delbaen's paper, or Delbaen I'm not sure how you pronounce his name, only seen it written up to now. I want to really get into that, because I did pull it up, during my preparation for the show. And I had a look and didn't get very far, in one evening after putting my kids to bed, which is usually when I do research for this podcast is not a good time to be reading a very remarkably dense mathematical paper by by some guy in some university in Switzerland. But we're not gonna do that yet. So the the thing that I want to pull out of that, and I'm going to hold you guys to this question of what we hope what you tell people at a dinner party you do for a living. But the interesting theme that came out of that, you know, those comments, there was, weirdly, actuaries aren't even really sure what they're doing or sort of how they're doing it. Right. Or it hadn't been for a long time, you know, because we have a lot of the sort of insight into how actually what we do works or how it should work, buried in a paper that was written not that long ago, and actuaries have been around for quite a long time. And so to me, it's like, I felt this a lot as well, where it's actually quite an, you know, we don't people in the insurance industry generally don't fully understand, kind of why what we do matters for people. And how it does, because it really does. But we're sort of all kind of part of this industry that just sort of works. And we don't have a lot of kind of meta insight into it.
Steve Mildenhall:So I would respond that I think, for many years, it didn't matter. Right? So [interesting]. So you go back and look at how risk loads were added into insurance prices, particularly in the US. There was this thing called the commissioners decision that I think happened in the 20s or the early 30s. And it basically said underwriting margin of 5%. That's it right. We're done. And everyone just was happy. And they went on their way. And they loaded that in. And there wasn't a massive variation across lines of business, I think fire had a conflagration a cat load in the in, you know, olden times more like the Chicago Fire, right, a massive fire in a city as well, people worried about, you got an extra point or two for that. But it was basically five points across the board. And it wasn't sort of a whole lot of variation. As John said, that sort of Andrew was such an important event for the industry because it was like, Whoa, all of a sudden, we've got, you know, State Farm fire and casualty just lost every penny made since inception in 1935. Or whenever they lost in Andrew, right, a very large, very old established homeowners insurance company. This was a complete game changer. And, you know, what, went with that sort of massive increase in risk was a massive increase in risk loads. And so all of a sudden, rather than talking about whether is it 4% or 6%, which you know, probably deserves a three page paper because this is like, I don't care. It's a bit of a yawn. Have you now now you're talking about buying reinsurance that has a load of, is it 2x 3x? Or 5x? Right. And it's a much bigger deal. So I think it's, it's, you know, there's that for the modern era that starts with CAT modeling and what have you, I think it's sort of starting it was 30 years ago this year, right, that, that Andrew happened, it's a bit unfair to sort of characterize this as blundering along, it just sort of wasn't an issue until these cats started happening.
David Wright:John, anything to add to that
John Major:we're, you know, you start there, and you look at how people have been studying it. And there's been a lot of different directions and a lot of drift and a lot of blind alleys. And we can spend a lot of time talking about the blind alleys. Don't want to get into that quite yet. So yeah. So that's to tagging along what Steve just said, Now, he want to talk about what I do for a living. You know, what actuaries do for a living all that, you know, that's like asking what engineers do for a living? It's, it's, there's quite a variety there. You know, this, this particular actuary, I'm not even sure I've been acting as an actuary. I've been doing research, I've been trying to figure out consistent, sensible models for how this little corner of the financial world works.
David Wright:Figuring out what actuaries should be doing, you know, your point, Steve there about the history. That's a brilliant point exactly where I wanted to go at the my thought, tell me what you think about this? Yes, Hurricane Andrew, obliterated, you know, Florida insurance companies, or 26 of them or something crazy, like that went out of business. Right. And that
John Major:Two years later the Northridge earthquake.
David Wright:And then Northridge
Steve Mildenhall:18 months, 18 months or 14? Not less than. Well, less than two years.
David Wright:Yeah, that is pretty amazing. one two punch to the industry to wake folks up. But my thought there is, these are these matter as a as a it's a, it's a question of scale relative to the industry, I think, I mean, relative to expectations, the industry, but also relative to the industry as well, because I think like, if you go back, I don't know, 300 years, 500 years, 10,000 years, whatever it is, whenever it kind of the concepts of insurance are kind of permanent, I think part of social culture, I think to one of your original points there, Steve, the scale of a disaster is and they've always been very large. And the shock of how to handle or so the shock of a disaster and how to handle it afterwards. Is, is in some way, the challenge of that is proportional to the resources you have available. Right? So what I mean by that is like in the 17 or 18 century or 19th century, large fires in a city would have been equivalent to an Andrew like, we will have had events like this in history, which today might not be quite I mean, I don't know the whole city burned down, I guess it would be bad no matter where you're from, no matter who you are, who you are. But I would think that like because the you know, the financial resources available to insurance companies in yesteryear were smaller, the industry is way smaller, there was no auto liability for one thing, which is a huge, huge proportion of the insurance industry. And so you had a smaller kind of collective pool of capital to fund risk, you probably would have had Andrew style, you know, assaults on the industry's capital base in the past. But we just didn't have the ability to do anything about that or think scientifically about how to handle it. Right. Is this what do you guys think about this concept that Andrew is sort of the next in the line of of a lot of disasters that have struck the insurance industry. But now we suddenly had the tools to kind of think through it. Think it through more carefully.
Steve Mildenhall:Yeah, I mean, the timing of Andrew relative to cat models, I think the early cat models came out sort of late 80s. Right. So there were cat models there, but I think they were largely not believed[they're ignored, right?] They were ignored. And yo, you did so you had the other big story out of Andrew, was PruPac, right? Prudential Property Casualty Company of America, that also blew up completely lost 100% of its surplus there. And that was a carrier that largely wrote risks in the Northeast. Well, an upper market kind of book. They all had summer homes in Florida![Yeah.] And they so they thought they had a northeast exposure. They had Florida exposure that they weren't really tracking quite as carefully or maybe maybe they were ignoring the numbers, but it was it was a giant shock, their market share loss was very concentrated around where New Yorkers went to retire in Florida, which was happened to be you know, we're Andrew hit. And so that was a fact that you know, as soon as that happened, we all realized, well, we could have known or that ahead of time. [Yeah.] And so we went from you know, Andrew was the greatest sales tool for the cat modeling firms imaginable, right? Hey, I told you so I was telling you so; it actually happened. Here it is. And it took, you know, it probably took five plus years because you had FHCF happened, right. Then you had Northridge, you had CEA, yuo had a lot of dislocation. And you had a sort of a complete transformation in the financing of insurance, right? Cat bonds came along the ability to do a startup. Standalone Bermuda reinsurers came along, there was an amazing series of innovations that happened kind of off the back of that. And a big part of that was the ability to measure risk, right to be to be able to come up with PMLs, to develop this language that everyone kind of used interchangeably. Rating agencies, regulators used it, you know, cedents to reinsurers used it, reinsurers use it. Investors used it, everybody was speaking the same language. And it enabled the market to take place because people could communicate with one another, right? So I could think about how much capital I need, I can think about returns, think about how much reinsurance I need to buy. So it was a it was a wonderful sort of coming together of of the ingredients you needed to sort of really move risk management forward.
David Wright:So I want to just sort of interject there and just for people to people who might not be reinsurance actuaries listening to this, which we hope they will. Cat models were or vendor models invented in...
John Major:1960s, let me tell you the history of Cat modeling.
David Wright:I will ask you that John. Let me just sort of finish one more thought. I had an interview with Ted Blanche on this show, a couple of years several years ago, where he described in the viewpoint of a company which was much lauded as an early adopter and promoter of cat models. EW Blanch at the time, and he said it was the biggest disaster that being the investment into cat models in the 80s. He's like it was it was like I was I hated it. He's like, we're spending less money on this stuff. And nobody cared about it. And and it was just a pointless flushing money down the toilet. And then bang, the catastrophes happened. And now suddenly, people have this desire, just as you're saying, Steve, to bought to pay money to a software company to tell them what's the risk of hurricanes wrecking my portfolio? Remarkable. There's a lot to how a lot about human psychology wrapped up in that story. But John, please do tell us Cat models,
John Major:Dr. Don Friedman of the Travelers, he was a geophysicist and joined the Travelers in the 60s. And he was the first one to do portfolio risk assessment. Okay? The other geophysicists had been doing point risk assessment for some years. But he's the first one who said okay, well, what if you have a whole portfolio of these things? What does that look like? And so in the 60s 70s, and 80s, he was churning out these studies internally, for the Travelers; he was churning out papers for the scientific community, he invented cat modeling. And it was Karen Clark came to visit him and to learn, and she took a lot of this and developed it herself and commercialized it and made it into something that people would be willing to pay money for. Something that Dr. Friedman really didn't care about; at the time, he was a salaried employee. When when she became successful, the this whole group, this was in the Travelers, a Corporate Research area in the Travelers. They looked around and said, you know, we could have been doing this. So let's, let's do it. And they started their own little Natural Hazards Research Services, selling the same kind of services. And Guy Carpenter was their biggest customer. And long story short, that's how I ended up at Guy Carpenter.
David Wright:Amazing, quite a lot there about entrepreneurship. Right? You have and I occasionally work with insurance tech startups. And many, many times you come across the potential criticism of them, or what if the biggest insurance companies try to stomp you out? The answer is, well, they might come up with the right ideas, but that filtering through a big organization to turn it into a commercializable product, virtually impossible, I will say. And so it takes a startup like Karen did with AIR back then to.. and listen I imagine she had some lean years at the beginning there to Ted Blanches story about how it just wasn't working. It's nobody wanted it. So people in the Travelers are probably folding their arms being like, well got away with that one. We didn't have to do anything there. And then until suddenly, it was a tremendously good idea. So if you guys could cast your mind back for me to the pre Andrew world. What, what did actuaries do in these lines of business? You know, Steve, you're saying that there was no profit load, they must have I mean there must have been something.
Steve Mildenhall:It was it was 5%. So I'll have this one over to John, because I cannot pass my mind back to pre Andrew because I started in the industry on June I think the 29th 1992. So my pre-Andrew experience was about six weeks.
David Wright:Oh you spring chicken, you, Steve. Okay, John, tell me what were actuaries doing back then?
John Major:Experience studies. Just look at what happened and true it up and project it forward.
David Wright:So you one could argue maybe that cat models themselves are a version of experience studies, right. So they look at hurricanes in history, Steve, you don't like that one.
Steve Mildenhall:Okay, you could argue that right. But what I would counter with or add, maybe it's an it's in addition, is that unlike any other line of business, I could go away and I could build a model, that would probably be the right order of magnitude answer with no insurance data, because I can generate all the events for sure, with no insurance data, because it's got nothing to do with it. Right? Meteorology, seismology, so I can develop the events. And then I can put a house in a wind tunnel, and I can blow the roof off, or what have you. And I can do some studies to come up with some damage curves. So I think it's a really unique thing about catastrophe risk, is that actually, I can get quite a long way to pricing it with no experience. I don't believe you could do that with personal auto, I don't believe you could build a model that would say, Oh, I got all these cars flying around. And and, you know, what, when are they going to collide with one another? I don't think you could do that sort of cerebrally the way you can do it for cat models.
John Major:[And basically], maybe if you had enough Google location data,
Steve Mildenhall:maybe but even then it's not clear that you could really, I don't know that you get I don't, you couldn't, I don't think you could get an estimate of frequency. We know what the frequency of hurricanes is pretty well, right? We got that 150 years, I don't need any insurance data for that. I don't think you could guess that BI frequency would be 3% 5% 2%, whatever it is.
David Wright:Those are purely social phenomena. Right? They're just humans interacting with killer machines called automobiles. You know, walking around with AK forty sevens, and one of them one on one goes off by accident, you know, you're driving your car around. And, you know, one of the things I did want to actually ask you guys about we seem to keep hitting it over and over again. So might as well talk about it explicitly. Your book doesn't cover liability business, really. And now I want to ask your perspective a little bit because I was thinking about how to maybe you could adapt some of the ideas, maybe you have thoughts, but I mean, it is, you know, really very much about catastrophe oriented reinsurance, and property cat is not the only kind of catastrophe, you're gonna have catastrophes in other lines of business as well. But that seems to be kind of the core call it observation about the world that this has become a very important part of the insurance industry. And so this book designed to price it?
John Major:Well. The book says, if you have a probabilistic model of your losses, then here's what you want to do with it. [Okay] So you want to go ahead and build yourself a liability model that you believe in? Go ahead and use our book to get the risk load.
Steve Mildenhall:Yeah, I think, you know, if you can price, a multi line, you can do the allocations, I think the difference is, is that the confidence you'll have in your statistical model for the liability lines will be lower than what you have on the property side. Because typically, and we've probably all worked with people doing the, you know, the capital models and the internal simulation models. On the property side, you've run it through this cat model. And if you stacked up all the research, it probably reaches, you know, halfway to the moon or something. There's a lot of papers, a lot of scientists, and then you think about well, how did I calibrate that number for you know, my GL distribution? Well, an actuary went Oh, I think the loss ratio is about 68, the CV is 25% and I'm plugging in a log normal. Well, that's not gonna give you quite... And you got the problem with how you glue and all together with the correlation, right? So it's, there's some information there, and you can look at the tails and what have you, you know, there's, there's a, there's actually a lot of insurance information. If you go back, I've done a ton of work with that. But it's not really the same kind of quality as the information you've got on underlying cat risk. But the methodology is completely agnostic to line , it just it takes as input a multivariate distribution that pops out allocations for you.
David Wright:Yeah, you are. I mean, it is quite a general approach. All the examples are catastrophe examples in the book and I don't mean that as a criticism, because that's just the best application for the methods, right? I mean, by a long way.
Steve Mildenhall:And it's also the problem that certainly I saw everybody struggling with, right? I never went in and talked to management and have them say, Oh, I'm really struggling with deciding whether you know, like, do I like GL or commercial auto? I mean, maybe they don't like med mal. Some people have convictions against work comp or whatever, but the discussion around how do I balance cat against everything else I would say is almost omnipresent. Right? And it's a giant debate going on in the market right now. You can listen to quarterly conference calls and you've got see some CEO saying, we're pulling back we don't like it. We don't like the risk reward on it and you've got another CEO saying, Oh, we think, you know, we trust the science, we know what we're doing. We're experts, we're going to continue to grow. So you can see that debate like playing out in real time and it is it's a real debate and there's, you know, different points of view. And so it's just because the risk loads are so material that's that's why the cat space is so important here.
David Wright:What are so I can't help but jump on that oneI'll have tor write a note for myself to come back to this thread. But what are they disagreeing on , those CEOs? Fundamentally.
Steve Mildenhall:So when you listen to CEOs talk about volatility, I'm often they're really talking about realized volatility, they've had some losses. And they, and in that situation, it becomes very hard to disentangle the sort of potential for loss. The fact you've actually just had some loss and underpricing because there's nothing that makes it more likely for you to have a loss than if you've underpriced the business because you've just got less premium to cover cover the loss. So I think what's going on at the moment is, we had a really interesting time you go back, 04, 05 right, four event four events, Katrina biggest event in history - I think it still might be the biggest US wind event in history- happened. And incidentally, compared to Andrew, after Katrina, I believe the industry ran like 100.1 combined ratio, complete changing scale to change in ability to manage the risks and what have you. Not a not a giant disaster after Katrina. So you had Katrina, you then had the longest period in recorded history without a category three or greater making landfall in the US. So everyone got very happy with writing cat risk, right? And you can see it in the prices, the prices just went down and down and down and down, until you get to 2016. And then you had Harvey and Maria, and you have and then a bunch of like weird things, you know, freeze winter storm in Texas, and what have you, we just had Ian. And I mean, also. So it's been a sort of litany of, of unexpected, poorly modeled, catastrophe losses, that have happened since 2017. And partly, that's just sort of a regression towards the mean. But partly, I think it's climate change effect, right. And these models, the commercial cat models tend to be pretty sticky. People don't like their PMLs to change. They don't like having major revisions in the models. And so they're, you know, they're very much backward looking. And climate change is moving apace, right, I think we would hopefully all agree, the climate is just different than when I was a kid, right? It is cold is not so cold in the winter, and it is hotter in the summer, it just is it's observable. And how are we projecting that forward is leading to sort of underpricing. So that's another angle, you know, the simplest part of your premium is getting the expected loss right, we're talking about how you get that add-on for risk, but you've gotta get the EL part right. And that I think, is part of what's causing the problem on Cat at the moment, because we've got this trend is very difficult to see how to incorporate into your into modeling and your pricing.
David Wright:John, you have a take on what the CEOs are disagreeing about in these earnings reports.
John Major:No, I'll defer to Steve on that.
David Wright:One of the things that comes to mind, as you're talking there, Steve, this is back to what I wanted to at least finish covering on or not finished, but at least tie a piece to the liability discussion is covering... calculating a profit load is not the only thing actuaries do, or the actuarial function that an insurance company does, it is the only thing that you're covering in your book. But, you know, there's lots of other important stuff, things like understanding what the expected loss should be, and collecting the data and, you know, underwriting for that matter or figuring out whether the moral hazard of an insurance of insured or... and a variety of really important functions that create inputs to the pricing process. But those other inputs might be more important in other lines of business.
Steve Mildenhall:Yes, go first of all, where you've got to do very granular pricing, for example, and we're quite upfront about this, I think page one line about two or three is, you know, we start after the heavy lifting has been done right? We are definitely swooping in at the end so totally agree with
David Wright:And casualty being an important example where it's that. really the expected loss that's actually where all the work gets done, you agree with that?
Steve Mildenhall:It would depend what ca... GL is such an interesting line because it's so varied you know, ranging from your million primary on a some mom and pop you know, retail operation through you're writing excess D&O, on a Bermuda form for a fortune 500 Corporation. You know, the former is all about the loss cost and the latter is probably more about the risk load.
David Wright:Yeah, I would say like a premium weighted average across liability lines like mostly maybe primary liability, general liability for small commercial, I would think or auto liability.
Steve Mildenhall:It's more it's very much more loss cost. You're gonna get... So hey I think here's the issue is, whatever the premium load is, it's not going to vary, right? What separates property is I can know for absolute sure fact that your location is in Miami, and it needs a high risk load. And this location is in Dayton, Ohio, somewhere like that, right? It's very hard to do that for GL or commercial or work comp or something. So you tend to end up with risk loads that are sort of the same across the board, which then makes, you know, the type of work we're doing becomes less less important for those things.
David Wright:That's actually the thing that I wanted to kind of like, I don't know, challenge is the right word, because I think it is a little bit of a, you know, changing around of what you're trying to do with the book. But we do see volatility of results from the CEO and the earnings call perspective in casualty business, but a lot of it, actually, and there are periods of time, maybe just as many in history for volatile casualty results as there are for volatile property results. And, you know, in the work that, Steve, you and I've talked about, but I know John you were involved in this as well, you look back across history, it's the volatility of the casualty results that have actually really caused problems for the insurance industry as a whole as opposed to property. So in some sense, the volatility of casualty is a more important problem. But the source of that volatility is not the same kind of source. And so I guess you have to treat it differently from a margin perspective, like, you know, talk to me a little bit about why the volatility of the casualty results does not require an approach or in what way maybe it does require an approach similar to what you're describing in the book.
Steve Mildenhall:John you want to
John Major:I just say, it comes down to the modeling, you know, you gotta get a handle on that joint distribution of losses, you know, without that you're, you're at a loss. You don't, you can't you can't put you know, step one, figure out your loss cost your distribution of losses. Step two, okay, what does that imply for the margins?
Steve Mildenhall:Yeah, that's exactly it. And you can know that and have greater sort of differentiation in output on property, because it's location driven, than you can on casualty, right, there is simply no analog of a cat model for work comp, or something that's going to say, I mean, yeah, we all know, roofers are a higher... that it's a higher loss cost. And, you know, it's just not as kind of certain. And so it can't drive differences as effectively as it does on the
John Major:well, you know, some of my former colleagues were - property, so.. are - working on catastrophe casualty models. And you know, maybe someday they'll have some success with that. When they do, though, there'll be a comparable input, but they're not there yet.
David Wright:I'm still not happy. So I, because when I look at in the marketplace, I see certain lines of business that are kind of perpetually poorly rated, let's say, I mean, how many actuarial kind of presentations have you guys sat through, like I have at the CAS, that talks about trucking risk, it's like very hard to price for some reason. And some lines of business are. And there might be like, you know, social institutional factors, like, here's an example pizza delivery, right? So I work on pizza delivery periodically. Mainly, the risk in a pizza delivery operation is you have a kid, maybe somebody's not a kid, but let's say driving a parent's car delivering pizzas. And that is what they call a hired and non owned auto. Now, if you pull the hired and non owned auto product off the shelf, what that what that's designed for, is like a contractor that just uses the car once in a while to drive to and from a job site. pretty modest exposure. But this is like a, this is more like a Amazon delivery truck. Right? As opposed to somebody driving their car around, incidentally, you know, but the industry seems to like, get like fundamentally confused about what is what is going on here, because the label of the policy does not describe what they usually expect to see. And so there's like, you know, you'd be very wary when you're writing hired and non-owned auto, right? And, you know, to me, like, there's a, you might sneak in something, right? So you want to try to like, you know, what I'm trying to get out here is that the actuary might want to insulate themselves from some unknowables or some poorly known variables inside of the expected loss calculation through a higher margin. So I don't have the tools to understand this I know that the results are the pricing risk is higher here of getting it wrong. So can I not use pull up the pricing insurance risk book, and you know, calculate a better margin on on this because I want to protect myself.
Steve Mildenhall:So that's Volume Two. John is now despondent, because he didn't know there's gonna be a volume 2,
David Wright:It's a small book, it's gonna be easy.. six months.
Steve Mildenhall:So I was, you know, my goal going into writing this book was to do baby steps. I wanted a sort of rational, coherent model that I could apply to the sort of big problems that we see that made sense, right. And it's a simplified model, a lot of things get abstracted away. What I've subsequently been working on spend a lot of time thinking about is the kind of things you're talking about here, which is, it's really about qualitative risk, right? And so I've sort of coined the expression, I want a quantitative approach to qualitative risk, maybe sounds like an oxymoron. But where you get to with the examples that you brought up, is it tends to all be about the loss cost. It's, and yes, we could argue that it's, you know, there's more risk. So you maybe need a slightly larger risk load there. But But really, what you're saying is, you're not able to estimate the loss cost, or you're not doing a good job on that. And that's the problem; it isn't just, you know, in total for your line, there's going to be maybe 10 points of margin to play with your, you know, it doesn't give you the scope to come up with creative allocations that it does, on... you look at a cat reinsurer, they're gonna run a 50, 60 combined ratio, right? So you got 40 points of margin that you're playing with. And then you do expenses, what have you, as opposed to, you know, seven points. So I think all those things are important. Goodness of Fit, fitting categories is obviously is just kind of what you're talking about with the trucking and I love trucking as well, I think Commercial Auto is a great line of business. And it is almost universally, terribly done, right? You've got Progressive, I think runs about 15, 20 points better than everyone else, and they're growing. They're doing an amazing job and leaving everyone else in the dust, with a couple of exceptions. But that's that's broadly the case. But it's got nothing to do with risk loads. That's just because they can come up with the expected loss component more effectively. And they can do it cheaper, right? They've got this big, expense advantage, people tend not to look at that, but they've got an expense advantage as well. So they're pricing more accurately and they've got an expense advantage, but they are the future there clearly.
John Major:sounds like what you're talking about is not pricing risk, but pricing uncertainty.
David Wright:Okay. Could be what are the difference between those things, John?
John Major:Well, you know, one of the underlying assumptions or theories behind the book is, you know, why, why do we have these risk loads anyway. And, and part of that gets back to, you know, on the uncertainty, what you're dealing with. And we argue, in a few paragraphs here and there that the reason that high tail risk in cat bears such a large risk load is not because it's very risky, but because it's actually hard to get the loss cost, hard hard to get a good handle on the distribution up in the tail there. So it's really the uncertainty that's driving the the margin. And maybe in Volume Two, we can expand on that and see, see how it might affect other lines of business?
David Wright:You know, that's actually you're touching on something I wouldn't mind if you could dwell on this for a second. So Steve, second ago mentioned, they have 40, or 50 points of margin in these deals. And that's the case. And if you just sort of walked off the street, and listen to us talk, you're saying 50% margins? Like, I mean, that's like Google gets 50% margins, what what could possibly justify other than an impenetrable network effect kind of your thinking or thinking about other typical business advantages? A 50% margin? Whoa, like
Steve Mildenhall:50% margin?
David Wright:Why is it 50%margin?
Steve Mildenhall:Because you're writing a very levered bet. And to make we went back to fairness at the beginning, right, you've got to have the financial resources to back your promises up. And if you promise to pay someone, say 100 bucks, if a bad thing happens, but there's only a one or 2% chance that it happens. The loss cost is, you know, so one or two cents, but you got to have that 100 bucks sitting there. And you might lose it, and you need some return for that. And you might want a 15 20% 15% return, say on that. So you need 15 cents of of total resources. So you'd be looking at, you know, say a three point loss cost 15% Premium 12 points, the margins, you know, four times you're expected to be even bigger, but it's simply because the bet is so levered, the tail is so big, and you've got to finance that tail, that's expensive. And the tail is only there because of the cat, you take the cat away, the tail goes away, you can run a much more levered book of business, you know in insurance terms, you can run it at a much higher premium to surplus ratio, and you can get a higher ROE with a much lower margin. So it's all about kind of squeezing the balloon, and cat means low leverage. Low leverage is
David Wright:Okay, so I want to get pushed back a little bit expensive. against the, you know, I'm gonna be devil's advocate here, and I'm going to criticize the entire project behind this book. And so I'm going to say that another way of putting what you said, Steve, and maybe John, you can respond to this first is, well, all the companies that don't charge a 50% margin go out of business, because they can't afford to pay for the thing that hits later on. Right. So there's like an emergent outcome here, which is the survivors charged a 50% margin. So that's what it's worth. And that's the, that's the market dynamic that allows the insurance companies to to continue offering policies because they are managed in a certain way. Now, the problem with that story, is that that's not a scientific process. You know, they could just say like, if you're like an old school Lloyd's underwriter I know, you guys know what I'm talking about. You're like, I just tried fifty percent margin. Why? Because it works. Well, why do you know it works? It just works. And he's right. Or she's right. And that's all they need to know, you just charged fifty percent margin in Florida, I don't need any of this Pricing Insurance, look at the size of this textbook, all this Greek symbols to hell with it, I'm just gonna charge fifty percent margin because I know that that works. And you know what? There's a guy charging a 40 and a 30 and a 20. And they go in and out of business over time. And eventually this this market outcome whereby we discover what the right level is based on the history that we experience. And so this is all just kind of justifying a result that we see that allows actuaries to tell themselves a nice story, but actually doesn't impact the market. John,
John Major:you know, I confess, I didn't really follow that logic. [No?] You seem to be arguing that that is the the way the world works. And that it's that it makes sense. And what doesn't make sense about that?
David Wright:Well, it doesn't make sense, because I'm arguing against the intentionality. So I'm saying that they have no control, you might say, I'm gonna have a spectral risk measure and spectral risk measures and all that later on this conversation. And I'm going to say, I want this kind of margin, that kind of margin, but really, you're just reacting to the market prices.
John Major:Okay. Fair enough. Fair enough. You know, and again, it's really kind of an input to the process that we're talking about, you know, our book doesn't say, Oh, you need a 15% margin, or you need a 40% margin. It really kind of starts with that, you know, in most of the applications that we're talking about, the required return on your portfolio on your business is an input to the process. It's [interesting] handed down from your CFO or you CEO, and the actuary doesn't get to choose that number. It's given to him. And so then the implications from there is that okay, if this is the hurdle rate for my business, then how do I apportion it out to the lines? How do I apportion it out to the policies, and that's where we spend most of our time talking about how to do that. Fairly and, and consistently, coherently. You know, the overall margin? You know, we don't we don't have a lot of advice as to where that's supposed to come from. That fair, Steve? [Yeah.]
Steve Mildenhall:No, I think, you know, just when you're talking about risk, it's a it's a qualitative thing. It's a subjective thing. There is no like, objective, right answer. What the market does is it puts a price on risk. And that's an input to us. And the reason that the margins are so, when we talk about these margins, let's be clear, here, we're talking about a margin relative to premium, right, not a margin relative to capital, that margin is essentially derived by people going out to the capital markets, and they're raising capital. And that's a very open, competitive, transparent market. And, you know, that cost of funding translates into these margins that we see on premium. But yeah, as John said, we're not, we don't have a view around what that right number is, and you can see it, it ebbs and flows over time with the market. And that's what we've seen in the cat bond market, you know, recently, it's gotten a lot more expensive. And so yeah, that's it's an input. It's not a it's not something that the theory derived. But as John said, what we're focused on is Alright, so we've got this number, this, this total margin, you need to allocate fairly between your insureds and the implications of you don't want subsidies and what have you, when you do that, that's really the sort of central work of the book is to come up with ways of doing that.
David Wright:So one of my, my favorite passages of the book is, well, you reference it at the beginning, maybe even this words at the beginning in the intro, where you say, you said, you have a lot of really interesting kind of encapsulations of kind of why you wrote this book. And one of them is, okay, what's the what's like the, what's the TLDR kind of version of this book, which chapters should you hit, you have this list, you're saying, You should only read these and you list out like, and it's probably a 15 bullet point list, be like this section of this chapter in this section, this chapter. I love that and I did that. But I also meandered a little bit more broadly, but there's one part you say read, you know, if you have to calculate a risk margin, read this section and then read it again. And then read it twice. And I read that section more than twice. And what's interesting about that section is that you start by talking about the regulations around actuaries the statement of principles, god, what are ASOPs now? Actuarial Statement of Principles, right? Sorry. And then, and then you say, and then look at market prices. Look at the bond market. And I don't know about you guys, but I don't know if there are many actuaries who spend much time looking at bond prices. But this is what you're getting at, right? You're saying, Well, what is the market price for risk, and we have to go to markets that trade in things in a liquid fashion to get sort of get an accurate ballpark estimate for certain probabilities, what they pay with their, you know, how much they pay in some marketplace to be able to translate that and there's going to be some judgment and other things you apply. But bringing that back to insurance, but I was consistently thrilled, this is one of the reasons why Steve, you and I get along is that you do and you know, mentor to all of us, Don Mango, just talk a lot about kind of, we're embedded in a world around insurance. That has a lot to help us with. And it's not just the math papers, some guy in Switzerland has actually there, there are lots of analogous processes in the economy that insurance actuaries can and should and must! learn from.
Steve Mildenhall:Yeah, I mean, so on that, I'd say, probably, certainly, one of the more important ideas in the paper, is when we think about a cost of capital, Is it constant or not. And the evolution of that is quite interesting. So you go back into the 80s, and the 90s. And people were doing this underwriting betas they were trying to find systematic risk and underwriting yo, because we wanted to use a capital asset pricing model type thing. So if a lne had a systematic component, you know, it did worse when the economy did worse then that would be more expensive line and blah, blah, blah. So we tried to do this, and it just didn't work. And it was terrible, in the sense that there wasn't enough sort of statistical information to come up with a clear signal. And the academic papers at the time said, we all know that the cost of capital should vary with line. But frankly, trying to estimate what what the variation is will introduce more error than it's worth. So we'll just go with a constant cost of capital. And that was sort of
John Major:that admitting admitting that it was wrong
Steve Mildenhall:admitting it was wrong. Now, as soon as you've done that, a number of things become really easy. Because essentially, when you do, you're talking about allocating margin, if your cost of capital is constant, allocating capital and allocating margin are the same thing, because you just take the capital, you multiply it by constant, right? So it's a super easy thing, and you get all of these nice sort of marginal capital allocation methodologies come out. Now, what we somehow forgot there was, well, that's one dimension, we could think about where the capital is constant, right across line of business. But there's another dimension that we can think of, which is how far removed from loss is that dollar of capital. And if we had thought of that, we could have opened our Wall Street Journal on literally any day it was published, and we kind of go somewhere to the back, and we could have found a credit yield curve, that would tell us what the rating was on triple A rated corporate bonds, double A single A triple B, blah, blah, blah, and what do you know, we would see those yields are all different, right? That the further removed from loss you are, the cheaper your capital is, okay. And any within an insurance company, you can think of that as right. So I've got this big slug of capital supporting my risks, the dollars at the top end are cheaper than the dollars at the bottom. Alright? So in terms of the sort of required return, and if you could sell each dollar separately, there, you would get a you know, a higher price less discount at the top, then you get at the bottom. Now, whether it becomes super, super important is the consumption of the different layers varies across the lines of business. Okay, so as soon as you allow your different layers to have a different cost, which they incontrovertibly do, right, every single market in the world, do you see that: cat nond market, corporate credit, you know, sovereign credit, everywhere you see that. Because the lines consume different amounts of capital by layer, they're all going to come up with their own unique cost of capital. So it gives us a sort of backdoor way to figuring out what the cost of capital is by line.
John Major:Okay, I think, you know, historically, what the big problem was, is that once we started to you know, once securitization got interesting, capital markets started getting interested in insurance insurance start to get interested in capital markets. All of these researchers, academics and whatnot, people writing papers, they focused on capital allocation. And that was the big diversion, the big red herring, chasing capital allocation. And and thinking that if we can get this problem solved, then all we have to do is multiply by the cost of capital, and voila, we're allocating our costs our our margin. And, and just, you know, how many papers were written to allocate capital? I can't even count them.
David Wright:So John, you said something pretty interesting there. That is a massive detour, intellectual detour the industry took in trying to allocate capital. What do you mean by that?
John Major:Okay, you know, I'm not sure the genesis of it, I think allocating capital seemed to be a natural thing to do. Because if you're a finance person, all of your studies have been with industrial companies. And allocating capital makes sense there because, you know, a dollar to buy a new equipment or a factory or something is a dollar that you cannot spend somewhere else. And so, you know, capital is allocated, that's exactly how you do it. And you have questions about the the NPV of the project, et cetera, et cetera. And so that mindset, I think, carried over to the insurance world, where they said, Okay, well, you've got all these different lines of business. And so let's, let's figure out what's the right way to allocate capital, among them. And, as our fearless leader, bless his soul, Don Mango pointed out, you know, in the insurance industry, capital is a shared asset, it's not the same, and therefore, doesn't, you know, it's not immediately obvious that allocating it is the right the right thing to do. But nonetheless, that was the approach that was taken. And of course, in academia, in order to get published, the safest way to get published is to start with something that someone else published, and improve upon it. And, and so, you know, once you get momentum in a certain direction, it just keeps going. Now, I, I'd like to tell you a little, little personal story here. And I want to preface it by explaining a little background in my association with the National Bureau of Economic Research insurance project group. When, when Guy Carpenter started getting involved in securitization, we hired Professor Ken Froot of Harvard Business School, as a consultant to help us work those things out. And one of the things I was looking at was the basis risk of index linked contracts and it turned out, you know, I turned that into a paper presented at a conference. That's actually turned out to be I think that was the inaugural meeting of this group and eventually accumulated quite a few of the demigods of the insurance economics profession. You know, names like Dave Cummins Ken Froot of course, I got a bunch of names here, if you're interested, and they started meeting every year, up in Cambridge, and I was privileged to be able to attend those meetings too. Even got to present a paper a couple of times. But the whole point of that was, I was there when Stewart Myers presented the Myers Read capital allocation paper to the NBER meeting there and the reaction, my reaction, and I think other people's reaction was pretty electrifying. It's like, after years of people groping towards how to allocate capital, some methods looking pretty ad hoc, some of them not so ad hoc, but not perfect. It looked like they found the answer. And, to me, it was electrifying. They had, it was almost like a magic trick. It's like this, you know, take a few reasonable assumptions and work it through. And in the end, you have something that actually is an allocation that adds up. And, you know, it wasn't obvious at the beginning that it was going to add up, but by golly, it does. And, you know, I ran to the phone to call my boss, Gary Venter and tell him it was solved, you know, the capital allocation problem is solved, you know, back then, you know, I was still drinking the Kool Aid too. And, of course, you know, it wasn't the last word. And I'll let Steve,
David Wright:Whoa can you tell me if you remember before we move on, and maybe this is where you're gonna go. But what was the insight? What was saying that cracked it? Well, I mean, fully understanding this is like a tangent for the business. But what was the thing that they did?
John Major:I think I'd like to defer to Steve, okay. He's got a better handle on the ins and outs of this than I do.
Steve Mildenhall:So that the tangent is the right... the critical word there, in what you said. The Myers Read allocation formula relies on the fact that if you've got a straight line, you can get to anywhere along the straight line, you're the tangent point at anywhere along the straight line is the same. Right? The tangent point always points along the line, there's no curving going on. And that's why Myers Read works, right? Because it's basically saying I can extrapolate as far as I want along a line because it's straight. And the problem is, insurance losses are not straight in that way. Because they might... What Myers Read relied upon was that the shape of the distribution of losses doesn't change with volume. Well, it manifested does change with volume, right? Because I've got one vehicle that I insure I have a 90% chance of no losses, and then some losses. If I've got a million vehicles, I insure I've got essentially no chance of no losses. And it's a completely different shape. So that assumption just doesn't hold in practice. And that assumption that your your distribution, or the shape of your distribution is invariant to volume, is this, the key thing that Myers Read relies on, right. Now, why did they make that assumption you might ask? Well, because, again, going back to what John said, that is the correct assumption to make when you're dealing with assets. When you're dealing with a portfolio of stocks, each stock has got a return distribution, and the distribution I get from owning 1 stock, 1000 stocks or a million stocks of one company, it scales exactly, it never changes shape, right? It just you just multiply by the number of stocks you've got. So that is the exact right assumption to make in the investment world. And as usual, the investment guys don't that that doesn't apply to insurance. And you know, we got a long way with doing right. So that's not, you know, all due respect, right. And there were a lot of good insights came out of that. But that is not how insurance works. It doesn't apply to insurance. So it actually wasn't a magic solution. Although the idea of a marginal approach, you know, essentially what we ended up with and is okay. Again, provided you're differentiating, you're taking margins of a differentiable smooth function. And that is just a little detail that often comes to bite you in the butt.
John Major:So you can see the mechanism right here, okay, you can see how somebody like Steve can look at what they did, and put his finger on the problem. And if he cared to at that time, he could write another paper that goes into that and explains how that needs to be adjusted to do a better job of capital allocation. But everybody's drinking the Kool Aid. Everybody is assuming that allocating capital is what we ought to be trying to do. And that's the big mistake. So
David Wright:which he did.
Steve Mildenhall:I wrote two two papers about that, got a grand total of about six citations. But you know, you try.
David Wright:I read them, Steve. And I can't say like I put enough effort in to fully grasp them. But I do want to come back just for a second to kind of this concept of, well, just a little bit of this story. So what appears to me that they did was they took a result, which was well established in capital allocation for equities, and then just copied and pasted it, I mean, more to it than that, but imagine if you're Myers, or Read, and you're you're wondering, I know, this is a problem in insurance, but we just figured this out. So guys just do this.
John Major:Nah, it's a little more sophisticated than that
Steve Mildenhall:There was. It's interesting when you read the papers, that it's always easier to explain something, once you understand it, as opposed to develop it in the first place. Right? It's like the people who invent something take forever to do it. And then the people who copy it, do it really quickly, because they've all the all the problems have been sorted out, right? So do and there was we've got a whole chapter basically on the sort of the struggle to get to a consistent theory, and it's a really interesting story. So, you know, it was a good paper, it was a really important stepping stone. And
John Major:if I recall correctly, if I recall correctly, it was really about equalizing the insolvency put, wasn't it?
Steve Mildenhall:Yeah. EPD. Yeah. Yeah.
John Major:So you know, it's not just a simple extrapolation, like CAPM or something. It had a little more nuance to it than that. [Okay.]
Steve Mildenhall:Let's carry on if we could, I just chomping at the bit to say a few things about this allocating margin versus allocating capital[Please do.] So as John said, for manufacturing, capital gets spent so it's you've got a pool of money or you can raise money but you spend it; it goes somewhere. It sits in plant and equipment. And it's obviously it's a pooled resource for insurance, it's different, as Don pointed out. Also, I would note as one of the really other important papers here was the Phillips, Cummins and Allen paper that came out around the same time 96, 97, where they use option pricing theory to do an allocation, and they explicitly say, capital is a shared asset, it does not make sense to allocate, right, we're trying to get to prices. But if you compare thinking about allocating capital to allocating margin, and the way you've got this margin that you want to allocate is, you've got a certain total amount of capital. And you can look at your peers and things and you can look at valuations. And you can say, well, I, what number do I need to publish the Wall Street to, you know, have a decent valuation? Okay, that's the amount of margin I need to make, right? And you've got to then think about allocating that out across your risk sources. Compare that to allocating capital, right? So you publish detailed financials that give you the components of the margin, they are real, tangible things, business unit leaders are held accountable for that
income that they produce:margin, right, this is what it is, it's a real thing. Your you have stock analysts who are estimating how much margin you're gonna make, right? There's a lot of people publishing real and you can at the end of the day, you can point to the dollars, there they are, there's that there's my margin. It's a real thing. The capital allocation [is] completely not, completely notional, right? No one has an opinion on it. It's not published anywhere. There's no sort of external views written, it's not a tangible thing. In the way margin is. So just simply from the fact of, of corporeal versus ethereal, there's a big advantage there to allocating margin. It also matches up really nicely with risk, right? You think about what does your income statement look like? Well, you've got underwriting income that goes with underwriting risk, and you've got investment income, that sort of goes with reserving assets and reserving risk, and capital and asset risk, right. So you can sort of match things up a bit better. You never have this problem, which comes up often when you allocate capital, oh, we've got excess capital, what do we, how do we allocate the excess capital? Well, don't care. Because we're talking about the margin, if you've got access capital, maybe you need a lower return, it's reflected in the margin number, I'm just going to allocate the margin. So there isn't this problem, this kind of, quote, unquote, excess capital problem. And then but for me, the real, the real, you know, nail in the coffin of not allocating capital was I remember when I read Neil Bodoff's paper, which is another great innovation in thinking, and was really close, actually, to kind of what we came up with in the book with spectral risk measures. I went through that, I did his allocation. And ah okay, I see how this works. This is great, very clever. What's the premium? Oh, I don't know what the premium is. Because I need a cost of capital, about which he has nothing to say. So you do all this work to allocate your capital, and actually the thing you want, which is what premium, what am I going to quote on this risk? You don't know, because you're missing a giant ingredient. If you allocate the margin, you can determine the premium right there, because it's like, hey, I need $27 in margin, I add it on with the loss costs and expenses. I'm good to go.
David Wright:So if let me, let me try my understanding here. Okay. So capital allocation does make intuitive sense. Because in the case of your own physical capital, you can imagine dollars getting applied to a business unit, and then they have to then generate a return over those dollars. And you measure the dollars, you measure the return and it's all tangible, I get that. You also have a tangible amount of dollars in an insurance company. Now it sits in a pot, doesn't get handed to somebody, right? But I can imagine saying, you know, how much of that do I need to give you to show me what your return on capital is going to be? You know, I had a... I interviewed, somebody once, can't remember which one it is. This wasn't even on my podcast. Actually, this was like a panel discussion. I was leading somewhere. And I asked a CFO of one reinsurance company like what's the right metric for determining performance? And, and, you know, is it the combined ratio, or what is it, and he said, actually, it's a properly specified return on capital. That's what I want to know, I want to know whether this unit is giving me enough profit to fund the risk. And that requires a denominator and a numerator, the numerator is the amount of money you're spending and denominator is the amount of capital you have. So the intuition there makes sense to me. So Steve shook his head. Where's that wrong, Steve? And why does allocating margin give you a better...?
Steve Mildenhall:Alright, so the way the way I believe that the CEO should talk about it with a business unit head is I'm sitting here and I have $10 billion of capital, which and you as a business unit person are going to expose that $10 billion to risk and you could expose $1 of it, none of it. You could expose all of it, right? And I've got three or four other business units and they could also expose all of it, right? So, you know, I can't say oh, you only get a quarter of it. Because if you come to me and you actually have a loss, which is half of it, I am legally obligated to pay it. So what the CEO, I believe the better way to think about it is not, oh, I'm gonna give you a quarter of it. And so the charges is a quarter of whatever, it simply, there's an entrance fee to expose all of my capital to risk and for you, it is this many dollars. And I don't need to go through this extra step of allocating capital. I don't, it doesn't add anything. It's it, it, it's an historical and it goes back, as John said to thinking about manufacturing products and IRR and NPV and all that type of thing. But you don't have to do that, you can just do it directly. And you can come up with a view of: all right for you, it's 250 million or whatever. And for this guy over here, it's 350. And for this other guy, which has virtually no risk is only 50 million, I'll give you access to all 10 billion for a much lower number, whatever it is, but you can do it directly as a sort of gate charge to access all of your capital.
David Wright:Okay. And that gate charge,
John Major:let me say in a slightly different way that the CEO says I'm sitting on a, a billion dollars of capital and exposing it, all of my lines of business and I have determined that in total, I need $100 million of margin to cover this risk. You have to get that, that's that's what my investors need to see. And so I need to take that 100 million and gather it from all of you guys. And given the risk that your line of business presents to this pot of money,your share is 25 million.
Steve Mildenhall:Yeah, exactly.
David Wright:Brilliant. So how long did it take us to get there? So we have Myers, let's get back to Myers Read. That's Myers Read right. Now, we sort of get a feel for the endpoint. What tell me more about the journey. I want to hear about the history. I think the history is really interesting.
Steve Mildenhall:So I think all we go through like what I would sort of regard as the, the seminal papers here, right? And it was Myers Read, there was a Shaun Wang paper. There was the Philips Cummins and Allen that we discussed, there was a paper by Sherris. And then there was Ibragimov, Jaffe, and Walden, that was I think, 2010. I believe they all have a constant cost of capital, a common thing, they're all tucked into this capital allocation framework. I'll let John talk about Don coming up with it's it's a shared asset. And I think didn't Gary Venter also talked about allocating margin. But for me, the penny dropped when I realized that the cost of capital varies by line. Now, you can't interpret an ROE anymore. Because if you're constant, if you've got a constant cost of capital, 10%, and you return me 12 on your capital, that's good, right? It's objectively good. And if you return me eight, that's objectively bad, but if my cost of capital varies by line, because my capital cost varies by layer, different business units consume different amounts of capital by layer, so you get a varying target. Now, I don't know if 12 is good. 12 is bad if you should be returning 20. And it's great if you should be returning 10 I don't know. So it's kind of a useless metric.
David Wright:So John, maybe you can maybe be good. It'd be interesting to talk a bit about some of the folks that Steve just mentioned in passing, because you got to work with some pretty awesome thinkers within the insurance industry at Guy Carp over the last 25 years or so.
John Major:Yeah, well, that. Yeah, I'll get to that. But let me let me start 35 years ago, when an Israeli economist named Menachem Yaari looked at utility theory and said, you know, there's another way you can do that. Now, utility theory, as we know, takes takes a distribution, in our case distribution of losses, and says, Okay, well, we're going to
transform money into utility:utils. So we're going to have a function that takes a dollar and turns that into what that dollar's really worth to you. And then instead of taking the expected loss, p times x, we're going to take p times utility u. And we'll call that your expected utility. And so you have this whole Utility Theory built up around that principle. And Yaari said, Well, you know, what if you left the dollars alone, and altered the probabilities instead? So it's kind of a dual to utility theory, and that's what he called it, he called it dual utility theory. And he built up a whole framework of that, that was more or less equivalent in terms of power and what it could do. And so if you if you look at that, what is that the expected value of a distorted probability times the actual loss. That's what spectral risk measures do. They take this distorted probability, and use that instead of the original probability. So he, he did this 35 years ago. And there it sat out in the academic literature. And so then there was Shaun Wang and Gary Venter. I was fortunate enough to work for Gary Venter for a while, and to meet Shaun Wang as as part of that, and I forget which one came first. But Gary wrote a paper that said, Well, you know, if you wanted to price, reinsurance, so that it was internally consistent and arbitrage free, what you really need to do is you need to do something that you can get it down to like the individual dollars, what is the cost of a Bernoulli layer and a layer that pays off all or nothing, and you price that and therefore, you can then price anything else, by adding up these a suitable weighted average of these layers. And only if you do that, can you be sure that you're arbitrage free, you know, it's sort of arbitrage free, but you know, arbitrage free within the context of your own pricing structure, and Shaun Wang, formalized that, and came up with the application of distortion functions to pricing insurance risks. And so that, you know, between the two of them, this was this was really what kick started the understanding in the actuarial literature that this was something you could actually do. You know, at that time everybody's casting around for risk measures, what's the right way to measure risk and a risk being a distribution of losses is a big, complicated beast, how can we boil it down to one number, you know, what's the right way to do that, and they're just a virtual zoo of different ways of approaching that; different functions, different families of functions, and this was one family of functions on how to do that. And now that, you know, if you can follow that thread through that's, that's what we ended up with, in the book, pulling that thread out amidst all of the noise of things that did it other ways.
David Wright:So I do want to like, I do want to kind of ground this in a bit of your sort of like lived experience of being going through that moment. Because I think you had a great it's a fascinating thing that a reinsurance broker, first of all, it doesn't take any risk, employs a bunch of research academics who are actuaries which is you know, you mentioned, John, that you never really practice too much as an actuary, you mostly are a researcher. But you were an actuary and are and, and same with Gary. And Don came a little, little bit later. Rodney Kreps, right, so there's a bunch of these people who are employed at Carpenter, again, not a risk taking institution, but doing really fundamental research and influencing the direction of the industry from this place. I'd love for if you could just reflect on what that was, like early on, and sort of, did you ever kind of have a sense that you guys were really doing real good bread, groundbreaking kind of work?
John Major:Well, I'd like to believe so I like to believe I wasn't just spinning my wheels. You know, back in the, you know, again, securitization started in cat bonds became very important and, and popular. Pricing cat bonds became a big issue. How do you do that? And, you know, so we're brokers. We don't take any risks. But we got plenty of clients who do and who are coming to ask us asking these kinds of questions. So it behooves us to try and come up with some answers. I remember, Rodney and I did a tour of reinsurers, we went down to Bermuda, I talked to a bunch of people there, upstate New York, we went and we went around, and we sat with their actuaries and we asked them, can you what can you tell us about how you price cat risk? What do you what are you willing to tell us and they were surprisingly forthcoming with explaining, you know, what they did, what they knew how to do, what they didn't know how to do. So we put that in a paper along with a regression analysis I did of some some layer data, reinsurance layer data that we had accumulated. And that became a chapter in Morton Lane's book Alternative Risk Transfer. And I think that was probably the second paper to appear, explaining how to price cat bonds. The first one, Morton Lane did himself a few years earlier than that, and then there's a whole a whole slew of those that have come afterwards and they're still doing it they're still coming out with, I just just saw one today on ResearchGate another yet another paper on how to price cat bonds. So you We thought, you know, we were, we were groping towards answers there. And this was something worth doing. And... at one point, my goal was to come up with a credible way of assessing and estimating the market value of risk to an insurer. Because we needed something like that, in order to tell you what reinsurance is worth. [You're trading it.] What's reinsurance worth? Should I buy reinsurance? How much? What kind should I buy? And it's like, okay, so the standard answer back then was, well, you got your cost. And you got your risk. And so we'll do a two dimensional plot, and put scatter points of all of your options here and identify the efficient frontier. And so you know, whittle down your infinite number of possibilities to a finite number of possibilities. And then the client would say, Okay, well, where do I want to be on the frontier? And we're like, Well, where do you want to be? Let's, let's sit down and get your risk appetite figured out. So I wanted to collapse that down to one dimension: value. And my first attempts at that were highly sophisticated and highly ineffective for a couple of reasons. One, too sophisticated, hard to explain. Don Mango got me to simplify that down. And then it was easier to explain, but then it foundered on the second reason, and that was because cost of capital was an input and it was assumed constant. So it was only then when Don said, Well, think about this capital tranching idea of mine. When we started to turn towards this, we had a group that would meet in Norwalk, Connecticut, we called it project Norwalk, came from multiple cities, and even not even all the same company. Kent Ellingson was doing similar research on his own and Don invited him in. And we hashed through what might, what does capitol tranching really mean? And eventually came to the conclusion that it means spectral risk measures, it means distortion functions, it means what Shaun Wang was talking about years ago. And then, and then the the pieces fell into place. It's like, okay, this is the missing piece from my valuation models. And it appears in one of the later chapters of the book.
David Wright:So tranching capital and I've heard Don explain this bunch of times, we're talking about just having different costs at different levels of loss. Right?[exactly]. a worse outcome is worse. For me, basically, right?
John Major:A dollar that's at risk for a 1% probability of being lost commands a different premium from a dollar that's at a 10% probability of being lost.
David Wright:Yes. So now, Steve, I would like you to reconcile that with your earlier point about capital being cheaper, the farther away it is, from a loss. Tell me if I'm understanding and how do we put this together.
Steve Mildenhall:Yeah, so it this is the thing that is always blows people's minds a bit, because the further removed you get from loss, the cheaper the capital is as a percent of capital. But the more expensive, the insurance is a markup to expected loss. Okay, if you're, let's compare where you're at, there's a 50% chance of having a loss greater than that point and the 1% chance. So there's the 50% chance, you've got a 50% loss cost, and you've got to fund the other 50%, somewhere between premium and capital, right. And let's go with like a 10% cost of capital to just keep it easy in that layer. So you got, you've got to make five points of margin, and you're gonna get 45 points of capital, so your premium will be 55 cents. So your loss ratio will be about 90%. Right? So you're almost there. But you're still getting a 10% return. Now move up to the top, the 1% level, now you've got to fund 99 cents of capital. And you could do that with a constant. But let's say you're going to do that with a cat bond and maybe it's, you can get away with doing that at a 3% return. Let's imagine we're back in the days when interest rates were zero because I still haven't adjusted to that, you know, that'll shift, right? So you're gonna have to have three points of margin, and you're gonna get 96 points of capital, and you're gonna get one point of loss cost, but so you're going to 3% ROE, but what's your premium is going to be four cents and your last cost is going to be one cent, so your loss rate is going to be 25 cents or put the other way around your markup will be 4x, four times markup on expected loss to get to the premium. So the higher up you go, typically you get cheaper capital, but more expensive insurance because your leverage goes down because your premium to surplus ratio goes down.
David Wright:Right. So you need so much more capital just to sell like $1 Premium You got so you got two opposing movements. So it's cheaper but you need so much more of it that, you know, it's expensive in another way. How long did it kind of take? I'm interested in just for the evolution of Don's idea there about tranching capital. You know, we're where are we now? In, in time of the world history. So Kreps, no not Kreps, sorry. Myers Read comes out with 96, 95, something like that. Right?
Steve Mildenhall:Yeah. And reprint was even earlier than that. It's probably John probably saw it in the very early 90s.
David Wright:Okay, and so Don's idea of tranching capital where we start, we started thinking really hard about how there's not a constant cost of capital. When is that kind of happening? How many How long did it take for these to sort of emerge
John Major:10 years ago?
Steve Mildenhall:Oh, no, it was late. He was before 2000. Wasn't it Froot & O'Connell really was the first. That paper that you had the graph off that we use. Isn't that an O'Connell paper? I thought that was early 2000s.
John Major:Okay. Yeah, yeah. Yeah. I mean, well, you know, there's hints of that the all the way back to Yaari. So you know, what exactly you're you're trying to pin down here.
David Wright:I'm trying to like, one of the things I'm interested in is how it evolved the intellectual evolution of these ideas, right, because they come, they come in a certain order. And I think that it's nice to approach them in that order. While we're trying to well, I'm trying to learn about them here. And we're trying to explain them to the audience. And so like, you know, the kind of the temporal evolution of this these concepts, I just want to kind of trace that if we can, right. Okay. So it's kind of
John Major:like what you're what you're really asking for is the diffusion of these ideas into a broader audience.
Steve Mildenhall:So I think the chronology, I would suggest goes something like this. Prior to Andrew, everybody was looking for this systematic risk; you know diversifiable risk is not compensated. We want our systematic underwriting betas, and we're going to use a CAPM type model. And that's going to give us the cost of capital by line. And in the back of people's minds was the fact that well, that might be a little problematic for catastrophe risk, because that's clearly beta zero. So that would say that should be done a risk free rate. But let's let's park that. Right? Then Andrew comes along, bam, massive increase in the cost of cat risk, and you just can't ignore the fact that this clearly is not being written at a risk free rate of return, some giant rate of return. And so academics cotton on to this, and they're like, that's a puzzle that needs explaining. And so that was the beginning of this process, to say, All right, we need some alternative theories. And I, you know, it was late, late 90s. Early 2000, and I mean you've also got, like the Froot Sharfstein and Stein papers, right? There's reasons why insurers are what are called opaque intermediaries, right, they intermediate between the risk owners and the capital markets. And they're very hard for people to understand, it's very hard to see what's going on in the insurance company. And that means they have a high cost of capital, because you basically have to operate by trust, right? This is actually one of the other big innovations of cat bonds cat models, is they give you an independent third party view of the risk. You can't get that on liability, you literally have to trust the crusty old Lloyd's underwriters,"Oh I think the rate is you know, thus and such." And because you introduce that element of trust, that drives a capital trust component. And the fact that insurers have costly capital means they have a real interest in preserving, they don't want to get themselves in a situation, where they have to go and ask for more. Because the very act of asking for more will make underwriters think, oh, there's something wrong here. I'm going to charge you a lot for it. And you're going to get diluted, right? So they're all about have they got this sort of vested interest in risk management. And that's why it becomes effective for them to sort of buy risk, reinsurance and transfer losses and things. So I think it all went back to the big increase in cat prices that happened after Andrew that needed explaining it didn't fit into the existing theories, we had to come up with some alternative theories. SRMs was a great explanation.
David Wright:So love that your implicit reference to a podcast I did with Samir Shah on on innovating in the capital stack where the thing that I really took away from that conversation was just what you said there Steve, which is the the key to unlock a lower cost of capital is to give direct clarity right to the risk so now you don't have to trust people anymore but you're gonna have to charge for that trust with a higher cost of capital if I know what's going on and I can do it cheaper because I can I know how to diversify that against what and that's great, real profound point. I want to come back to this Froot O'Connell thing because you mentioned something in the book and if you already explained it to me in which case you can tell me this but they did a study was really neat of Guy Carpenter cat clients, or deals for a couple decades, I think, and they found a puzzle in there. And you guys guys have to sort of command here what that puzzle was that they were kind of like trying to figure out and and Solve.
John Major:If I recall the the notion was that uncertainty drives margin. But the but that doesn't make sense from traditional financial theory that uncertainty and risk should be treated symmetrically. If you have an upside and you have a downside, then it's the expectation that matters. I think that was the puzzle that you recall,
Steve Mildenhall:charging for diversifiable risk was the problem. That was a real puzzle to the, to the market
David Wright:so you have positive margin on on different regions. Right. So Florida hurricane versus California earthquake, and I'm charging lots of margin on these where I should just be charging a minimal thing, because that risk can go away. When you get
Steve Mildenhall:in investment speak, you have a zero beta stock with a big return. That's a problem for CAPM.
David Wright:Yep. Okay. That's right. And so what was the resolution to that problem?
Steve Mildenhall:Throw CAPM out. There, there are all these reasons that go back to informational issues about why capital is costly for insurers that do not apply in other situations, right, if I'm a Manufacturing Corporation, I'm going to set up, I'm going to raise capital to build a factory to manufacture widgets, I can compute the beta for that, you know, the cash flows that are going to come out of that particular factory, and it's all I've spent the money, you know, it's all very concrete and real, and it's not at all for insurance, I'm gonna sit here with a giant pot of money, I'm gonna have a, I'm gonna have invested in something which I'm probably not going to disclose to you very clearly, which is going to be a problem. And you're, you know, you're going to have to try and understand the risks I'm putting against that, which I'm not going to establish clearly, oh, by the way, we haven't mentioned reserving risk, which is a giant issue that comes along for, for casualty lines, I'm going to tell you my estimate of the losses, but I won't actually know for 25 years, you know, there's a whole bunch of things like this, that make it and I've got these regulator friends who can take over the company before it becomes insolvent, and take your money away. And they won't let me give any of the money back to you. But for all of these reasons, insurance companies are a horrible investment, right. And so they they command a higher cost of capital. And it's that it's that sort of frictional spread, that then needs to be allocated out, it's kind of a big part of what we're talking about here. And a lot and a lot of that is not pure risk, or pure risk in the sort of process risk sense. That's just additional risk that sits commonly across all casualty lines, when you get and then in addition to that, you get this pure risk element for cat risk, which is just the fact that you've got to finance a giant pile of liquid assets that you could lose at any any moment in time.
David Wright:There is another concept that I actually just checked my notes here that I forgot to mention earlier, but I think it might be helpful is is quite a lot of insurance is interested the idea of what the required return or rather like what the imposed return might be in a regulated environment. So you get the regulator, which we haven't talked about too much here. And there is a paper a long time ago, that was written that was about Massachusetts auto and that was Myers, Kahn. Yeah. Cohn. Okay. And so in that paper is really interesting quote about how I think it's in that paper, but what the required return should be for an insurance company a required return, but What the What profit insurance companies should be. And so a regulator is trying to say we're going to force everybody to buy auto insurance. As a result, we're going to try and prevent insurance companies from exploiting this privilege we're giving them to creating this giant business for them. So we're going to not allow them to charge too much money for it. We want to regulate the profit. So how much profit should we allow them to make and most of the conversation we're talking about here is in catastrophe lines, a little different from from from commer... er.. personal automobile, but I think the concept still sort of wind up getting invoked and catastrophe business where it's a regulated market. So how much profit should an insurance company make?
Steve Mildenhall:Well I cannot resist, you've brought up Massachusetts auto here, so I have to just go back and that was my, you know, intellectual coming of age, I guess, in the insurance industry was when John was working with these great minds at Carpenter, I was working at Massachusetts Auto, and I met Richard Derrig there who was the head of the Automobile Insurance Bureau. His name is on a lot of these papers. They had some conferences. There was a conference there where I saw Richard Phillips present his PCA paper with you know, which was a sort of competitor to Myers to the Myers Br... Myers and Read paper on the And interestingly, so Massachusetts Auto, they would do a rate filing, which was about, I don't know, 500 pages long. And then they would do the underwriting profit provision filing, which I recall was longer, it's like 800 pages long. And here's the amusing thing about this, that underwriting profit provision that those 800 pages justified was negative, because of the investment income offset, right, so it was to justify running at 105 combined! We needed 800 pages of quite abstruse math. Now, when you when you go through the Myers Cohn paper, it it doesn't actually deliver what you would hope, because it just says, let's take a cost of capital, well, your premium can only allow you to earn that cost of capital. [laughing] They would say, Well, you know, we'll often and the math guys would do the beta studies and try and come up with a, you know, systematic risk of underwriting. And it is plausible, right, the commercial or the personal auto, because it's, it, the amount of people drive is going to be correlated with the economy, and you've got the inflation effect, right, it is possible that that could be a non zero beta line of business. But But fundamentally, what that paper is saying is that you need the price to hit a certain return on capital, for it to be fair, but it doesn't tell you what the return is.
David Wright:So that return on capital is going to be given by somebody else, and
Steve Mildenhall:it's going to come out of the market, right. And this was the totally weird thing about Massachusetts auto was, every year they would have this exercise where they would go through, and they would find that there was insufficient competition in the marketplace. And as a result of that, the commission has set the rates, and the commissioners set the rates every company wrote at the same rates, and the same form. Right. And you couldn't underwrite, if someone came to you for insurance, you had to write them, okay, your escape valve was you could cede them into a pool, and then you would assume back some proportion of the pool. And they also had things like, there were deliberate subsidies built into the rates. There was another wonderful filing that the AIB did called the subsidies in the rates, they explicitly quantify what the subsidies were in the rates. So you knew. And if you had a 18 year old male in central Boston, I think the subsidy and the rate was like, you know, he was charged 1000 or 2000 bucks, and he should have been charged 6000 bucks, right, it was a huge subsidy. And it was all subsidized by all the drivers and non urban drivers. And it was all clear. So the pool consisted of all the 18 year olds, obviously in in Boston, and it was sexless rating, they were way ahead of their time, you could... the males, and the females had to have the same rate. So you know, there was the subsidies there as well. And it was an absolutely fasc... I mean, I spent the first I think three years of my career working, it was absolutely fascinating, because it was this sort of giant, iterative dynamical system, you had to guess; the game that you played was, what's my allocation back from the pool gonna be, right? And you could get credits, if you kept bad risks, but they weren't too terrible, you would get a credit against your assessment. So you have to figure out how much the credit was worth. Well, the amount of credit was worth would depend on how much business got ceded into the pool, because if no business got ceded, then it wouldn't make a loss, the credit wouldn't be worth anything. But if a ton of business gets ceded, it makes a huge loss and the credit's worth a lot. So the value that you have to assign to the credit depended on how much you thought other people would cede into the pool, while the amount they're going to cede then is what they think. So you get this giant iterative system, right? When you start off with an assumption to the value of a credit, you say, well, if everybody thinks this, they're going to cede this, and that's going to produce this other value for the credit and then you keep going around until you get to till you get to a point. And it was intellectually super. I mean, it was completely contrived, right? It was just a function of regulation. Because everywhere else in the world... Yeah, we all recognize we've got literally hundreds of insurance companies quoting on every piece of business; it's a really competitive market. But here it didn't work like that. But it was for me as a young actuary fresh out of college. It was fascinating I love working on Massachusetts Auto, it was great. I spent a lot of time talking with Richard Derrig about these things. You know, understanding all the work he was doing; he was really friendly guy. And yeah, it was it was it was a dream for a young actuary.
David Wright:So what do you learn? What's the lesson we can all take from that experience of yours? I mean, one there's like government control can yield some stupid things. So that one we can learn all over the place in this world, but from a from a from an actuarial perspective of pricing from a profit, capital. What is the in the theme of the conversation today that you take from I'd experienced that might matter for other parts of the business.
Steve Mildenhall:What I would say somebody I heard once described, they said, economics, you can summarize as people respond to incentives. And all the rest is just detail. And I think that's, you know, what I always try and do when we when thinking about an insurance problem is, what are the incentives? What are the motivations? What is the underlying economics? And then how is that going to drive how people act? And if you follow that, and you follow that first, that will generally get you kind of to the right place.
David Wright:Okay, I would like to talk about an institution that whose incentives are interesting to me and that's the insurance regulators. Now one theme that I don't think we've talked about yet in this conversation is this kind of cleavage between kind of a capital level that a company should hold, which is, for the most part prescribed externally, you have the AM Best and insurance regulators saying here's how much equity should hold. And you have a pricing process whereby you say, what's the required return now, to fund this capital you have to raise? And I'd like to talk about the regulator a little bit about what their incentives are, maybe you can start with that kind of framing on Steve or you could pick a different one or John, and how they, how do they think about this problem about how much capital insurance company should hold do you think?
Steve Mildenhall:So they promulgate minimum capital requirements through RBC and most companies run RBC ratio, I think the average RBC ratio is 700%. Right? So the people hold seven times more capital than they say you need. Because you mentioned rating agencies, the rating agencies actually are much more concerned with solvency than regulators, regulators are concerned with, where I started my comments, they are concerned with fairness, they are concerned that the premium on charging different risks is commensurate with the risk that they bring to the organization. And there's no unfair discrimination and all sorts of things. So I think, certainly in the US, I would say regulators seem to be much more concerned with the sort of fairness and the pricing side of things I think in Europe is different with Solvency II, the capital margins are much lower there. And rate.., rating agencies are concerned with solvency, I think that's actually been a problem, because the rating agency's incentive is not to be wrong, not to say this is a solvent company, and then have it become insolvent. And what does that lead them to do? It leads them to be very, very conservative. And that's why y'all they have these very, you could argue, I would argue, very onerous cat charges, right? So AM Best, S&P typically have a sort of stress test mentality. Not only do they want you to be able to pay a one in 100 loss, they basically want you to be able to continue writing business with no change in your plan after that loss. Well, that's a very different standard from a solvency standard, which would simply be: I just want you to be able to pay the one in 100 loss. And they just decided to do that on their own recognizance, right, that and that has driven a big increase in capital need. And it interests me that no one has picked up on, you know, who gave these rating agencies the right to drive such high capital requirements into insurance companies? And is that actually in the insureds' ultimate best interest?
David Wright:You know, I love that characterization. Steve, I saw that you made that you guys made that in the book, where you mentioned, how really the, you know, certain rating agencies, demand this incredibly high standard. Whereas you mentioned regulators, I don't know if you mentioned regulators, you mentioned a competitor to S&P and AM Best being Demotech. And Joe Petrelli has been a guest on the show a couple times. And I think Demotech is an underrated, if I may use that pun, organization, because they are they are at the very least introducing some competition into that business. I mean, not everybody, not everybody appreciates Demotech The way that I think that I do, or I want to, but man, at least it's a different perspective for crying out loud. And they do have a little bit more of this, you know, we're gonna make sure that you can pay claims kind of approach. And isn't that what we want? Right, but there's a debate, because if you talk to and, like I do, agents, all the time insurance agents, they tend to be concerned with stability of relationships, saying I don't want to have to go to to a new company every year, because I'm used to... in a lot of ways that margin of an insurance agency is built around not having to touch the business very much. Obviously, you kind of set it and forget it as people like to say, and even even customers that really want to touch the business they want to buy from insurance company, they want to just not have to worry about insurance ever again, please. So you could see you could see make an argument there that AM Best is doing more or less what the customer wants, even though that's not really what the regulator's concerned about is is that making sure everybody gets their claims paid.
Steve Mildenhall:I think you're stretching a little I think the customer wants to pay as little as possible for solvent insurance. And you're not giving them a choice. I think you're right. Demotech has introduced a choice. Right. And I think that's good. I think people would not ask, so would you rather have you know that your insurance company can survive this, pay your claims and continue, versus you pay less for insurance, and you might have to shop if they if they pay your claims, but they can't continue as a going concern? No one wants that.
David Wright:And yeah, you have the reinsurance business, which is, which has no equivalent to me, I guess, you do have the collateralized markets, right. So in the reinsurance business, the alternative to AM Best is nothing. It's like a bank account. Maybe, John, you want to talk a bit about that, you know, what about maybe how collateralized reinsurance works for a second, because you're there at the very beginning. And as an alternative to Am Best? Well, any any kind of reaction to that?
John Major:It's, it's all about having the money to pay your claims. If you're running a leveraged business, you've got exposures, big multiple of your assets. But you've got a low probability that, that you're going to blow through that. But if you want to be absolutely sure, then you limit your exposure to a certain amount, and you fund every last dollar of it. And then the question of solvency just doesn't arise. Unless somebody, you know, unless your counter... you know, unless you have problems with your counterparties. On your asset side, the money is going to be there. And there you go,[which has happened].
Steve Mildenhall:Which happened right after, you know, when the Lehman blew up, they blew up some cat bonds because of what they were doing on the the assets. So the other thing now, again, we go back to with collateralized, it works for property, because there's no reserves; it doesn't work for casualty. Casualty is once again, different because of reserves.
David Wright:Yeah. And, to me the miracle of casualty reserves, so and we can listen to the Samir Shah podcast, if you want, like some extensive treatment on this question. But the you have to hold on to the money for a long time. And if you have to capitalize, you have to capitalize a company that just right, so collateralized reinsurance, you buy $100 policy, it costs $5, they take your $5, they put that with $95 of their money into a bank account. And now you have $100 for your limit in case the claim happens. And so that is a you know, you know, at the point of sale, collateralized company, you don't need any rating agency, because there's no chance of insolvency again, as long as the bank is good for it. And Lehman was not where they put the money with Lehman in the 2008 crisis. But you can assume that away. And so you kind of have this, you have this world where every contract has its own bank account. And if you have you have a claims reserve that last 25 years, they're being asked to keep the money in it for 25 years. That's a long time. If you're looking for an annual return on that money. You can't really do that you have to diversify that, you know, radically against 1000s and 1000s of other bank accounts, who kind of only holding one. So the amount of leverage you get is huge. That you guys agree with all that characterization?
Steve Mildenhall:Yeah, I mean, that brings up something that I found interesting is one of our later chapters on reserve risk. And I think there's so there's a number of places where the way, the way we do accounting for insurance sort of gets in the way of thinking about risk clearly, because normally what accountants want to do is they want to match revenue and expense. Right. And so we we do that very simplistically, because we have an unearned premium reserve, right? And we say, well, we've got this accident year, the events will happen in the accident year. So we own the premium proportional across the accident year. And when you get to the end of the year, if there's any unexpired term, you know, we roll that over. And so that's that's the extent of the matching that we do. But what we should really be doing is and let's take the, you know, let's imagine we're working with either zero interest rates or we've discounted everything. Actually, you shouldn't earn the premium across the year you should earn the premium in the proportion that the risk is revealed to you. Okay, and it might well be that in the first year you're writing excess casualty, I'm booking plan at the end of the year there is zero variability about that it's going to take me two or three years to know anything, okay? And the you we tend as actuaries to conflate the emergence of information about a risk with the payment path. And we think that[interesting] a long payment pattern means, you know, the sort of risk carries on for a long time, but you can have different situations that emerge there, right? You can have something like work comp, where you maybe get into a periodic payment, and then you have an annuity type settlement, you're going to pay for a very long time. But you know very early on what the amount is going to be, if it's indemnity, if it's medical, you probably don't, because you've got medical inflation sitting there. And then you've got other risks, where, ah, actually the uncertainty exists for a very long time. If you've got some court case, and it takes me oh, you're going through discovery and what have you, you actually you're pushing the resolution of your uncertainty out a very long time. And we don't really sort of get into these temporal aspects. But they're very important in terms of sort of decomposing that ultimate underwriting risk and allocating it back to the individual calendar years that you go through.
David Wright:So I do want to say that we haven't yet covered maybe the most new sort of bit of jargon in the book for practicing actuary, which is this concept of spectral risk measures? Certainly was, I didn't hadn't come across that terminology until I started hanging out with Steve Mildenhall. And so I'd like you to maybe talk to us about what those are, what is what is the spectral, I think a spectral is like Ghostbusters, like the Spectre, which is like, maybe a James Bond villain, but it means something, something else. But I can't help but think about Ghostbusters, when we talk about spectral risk measures. That's probably what we're talking about. And, Steve, if you can give us a little bit of kind of background on these and tell us a little bit about where they came from.
Steve Mildenhall:So John mentioned this Yaari paper dual, a different way of thinking about utility, rather than adjusting outcomes, you adjust the probabilities, okay. And that's really what the fundamental thing of a spectral risk measure, it is a consistent way of adjusting probabilities. And what you're going to do is you're going to make the bad outcomes more likely, and the good outcomes less likely. And then you're just going to use those adjusted probabilities, take expected values, it's going to increase the mean, and you call that a risk loaded premium. That's that's sort of the idea. And what's interesting about that fairly simple sounding concept is, if you trace it back, it's throughout the literature in a number of different domains. So the Yaari paper was 1987, there was a very famous book by a guy called Peter Huber called robust statistics that basically had the definition of coherent risk, risk measures in it from a sort of statistical robustness estimation process perspective that was published in 81. The idea of adjusting probabilities to price goes back to the sort of no arbitrage pricing Black Scholes in 1973. And then if you go all the way back, and you're just looking at it from a mathematical perspective, there's a French mathematician called Gustav Choquet. And he published his paper on what we call capacities at the time in 1954. Right, so these ideas are, you can tell it's an important idea, because it emerges over and over again, in different areas of literature. So I'll let John talk about sort of the move forward from from Yaari and and kind of what we did with it.
John Major:The the, as we said, it's not a lot that's new and original in the book, we're distilling what's already there. But I would like to highlight something that is original in the book. And that is, Steve's what I call the envelope theorem. He wrote a paper called Similar Risks Have Similar Prices. And part of that is summarized in the book. And the basic idea here is, let's say, let's say I've got a portfolio, and I've got a price for the portfolio, margin for the portfolio. And I want to allocate that down to the policies or the lines of business or whatever. Now, if you have a spectral risk measure, a particular distortion function, of course, you can do that you apply the methods in the book, but what if you don't know your distortion function? What if you all you know, is I want to use a spectral risk measure to do that. But I don't know which one it is. Now, out of all, the possible spectral risk measures are not all going to work because they're not all going to give the right price to the portfolio. So there's a certain number of them an infinite number of them, that will give you the right price to the portfolio. Well, it turns out that you can identify the extreme ones among that infinite set. And you can examine them systematically and come up with bounds on what the prices on your components are going to be. I have a particular single line of business here I want to price and I can come up with a lowest possible price and the highest possible price among all of those special risk measures that will price the portfolio at at this expected amount. Now, that doesn't Sounds like a big deal. It's like, well, you know, of course, you know, of course you can do that theoretically. But the fact is, you can do it practically, that there's a practical way to approach that it's not computationally particularly onerous. And that's amazing to me that you can do that. And so you know, that that's featured in part of the book, and that is something new. Yeah, it sounds like something that, you know, of course, you know, theoretically, we can go through all of the possibilities, and there's going to be a maximum, there's going to be a minimum, you know, that's just calculus. But the, but it's more than just a theoretical result, it turns out that there is a practical way to do that, that you can computationally efficient way to do that, to find the maximum and the minimum pricing for every component of your portfolio, knowing only that it is a spectral risk measure, and it's pricing the whole portfolio a certain way. And that just blew me away. And I'm just so happy to have understood how that works. The paper is, of course, an academic paper and has to be written to a certain level of sophistication. So it, you know, maybe you want to start with the book as an explanation for that. But the other the other point is that that's if you knew nothing about the measure, except for the total portfolio price. Now, what if you know a little bit about that? What if, you know, a part of the distortion function, you know, say up in the high tail area where business is being bought and sold, and that you have some market information, but you don't know the rest of it? What do you do then? Well, we're working on that. Now. We're busting that, that problem wide open. And I'm hoping that it'll be part of Volume Two, or at least a paper,
David Wright:what, what is the insight
Steve Mildenhall:To expand on that at the same time, I've been using this in practice, it works, and it's useful. And people, I think, you know, they got a lot out of being able to see the ranges, they were able to make sort of selections from this was very helpful to be able to quantify what that range was and identify the components of it.
David Wright:You know, that raises an interesting point when I wanted to touch on, which is, you know, the book itself is tremendously practical, tremendously practical. I mean, there are a lot of very concrete examples, that exercises you work through, that I didn't work through. But then I'm not studying for the exam that this one day will be on the syllabus for maybe my kids. So and I also want to put a plug in for something, we'll put a link to his YouTube channel, there was a talk that you guys were a part of. And it's called ERM Diner, I think you call it John, we'll put a link up to that. I found that was really interesting. And there's spreadsheets, which I don't know if there's a spreadsheet or if I have access to that, but we'll make sure there's a link to a spreadsheet that you can if you want to walk through that series of talks and follow along with examples. For people who want to actually use this stuff, this is a tremendously practical application. I want to come a little bit talk a bit more about kind of the book itself. But before that, I do want to kind of finish this thought on that as you're calling it, John, envelope theorem. Steve, what is the insight that underlies the ability to determine bounds for spectral measures, arbitrary spectral measures if I'm understanding it right about what they might mean for the price of a risky process or an asset?
Steve Mildenhall:Well, it got it goes back to we've, we've mentioned the word coherent several times. And there's there's axioms for coherence. But one of the key axioms is convexity. And convexity means, you know, something is, if you draw a... join two points in the space, the whole line lives in the space. And you really want functions to be convex, they are very well behaved if they're convex. And in particular, if you've got a convex function, a local maximum is a global maximum. And so you're going to do optimization, you really want to optimize against the convex function. And that the envelope theorem is, is really just a corollary of the fact that you can see a certain space has to be convex, and then you can identify the corners of it, basically. And that gives you all the points that you need.
David Wright:I see.
John Major:Let me add, let me add, you asked what's the fundamental that's underlying it? And the answer to that is TVaR. [Okay.] Spectral risk measures are composed of TVaRs. And the extremes are very simple combinations of TVaRs.
David Wright:Yes, TVaR, tail value at risk. This is above a certain threshold, which you determine for the TVaR, you take the average of all the events above that threshold, right, the tail value at risk. Now I have a problem with TVaR, guys, since we're on it. Now, you brought it up, that I want to talk to you a little bit about because it really comes with an interaction between, I think the assumptions of this book, which is, you know, we're assuming the pricing is fixed, like sorry about the pricing, so we understand the expected loss All right, we are assuming that this distribution we've chosen is right. That said, the tail of all these cat model distributions are freaking made up. I mean, you might have one event that's kind of near the tail somewhere, that you have some real data on the last 100 or 200 years, but everything else are just like perturbations about events, you know. So for example, the one that I studied most closely in my career was the hurricane that hit Long Island, right, which is a big cat 4, Long Island Express, 1936, something like that. And then pretty much every really bad event in the in the models, the vendor models, RMS AIR, are variations on mostly that; a big strong storm, which have you studied, the specifics of the history is kind of really unusual, lots of ways you have to lows and things that shot through it really fast. So it stayed really strong, really late, and other kinds of weird things. And that's, you know, tail events are gonna be weird, but you kind of take that, and then and other events, which were not nearly as serious, and you're just kind of playing with the assumptions and those events, you are inventing events, right? So you are taking, you know, to calibrate a TVaR for a spectral risk measure, you have to assume the distibution is correct, the distribution contains a buttload of assumptions in it. And so I would say like, you know, if we're dealing with only TVaRs, we're kinda like, I don't know, you guys can push back and you think like, you know, the sort of taking that as a given is a little tricky.
John Major:Well, you know, the mean, is a TVaR too. There's TVaR across across the whole, across the whole spectrum. You know...
David Wright:Attaching at zero? Yes. Good.
John Major:Yeah. You know, we're talking about every spectral risk measure is a weighted sum of TVaRs, it doesn't mean they're all 99.5 TVaRs.
Steve Mildenhall:I got two points, if I could on this. One is, I think your characterization of cat models there is quite misleading, right? The reason that, so the cat, you've got these global climate models generate millions of events, not just hurricane of 38, we have 36, whatever it was, millions of events, right? Based in physics, atmospheric physics, and what have you. Okay, so I think you've got a pretty good spread of events. The reason that it's always New York, if you've been to you live in New York, there's a giant concentration of values there, right? It's always going to be in New York, I don't know how much value there is on Manhattan, but it's probably you know, it's just a big number. Always gonna be New York, okay. And then, you know, maybe it'll be Houston, it'll be Miami. But for it's like, I don't know, if you remember when, when terrorism models came out, the problem you always had was, you couldn't get a rate rounded rate that was greater than zero outside Manhattan, because there's just so much standing that is so close together. So that's the first point. The second point is John's John's excellent opposite reminder to us that the mean is a TVaR as well. And one of the things I've gotten, I like to listen to seniors... when you listen to like, on conference calls and things, what CEOs talk about risk, they're always talking about risk across the spectrum. And the spectrum of spectral risk measures is kind of the probability level right? So is it a one and 100 loss 99th percentile? Or is it a median loss 50th percentile? Or is it really good outcomes 10th percentile, the spectral risk measure is giving you your view of sort of how painful risks are to you across the spectrum. And there's a lot of... when you get into capital allocation work, what tends to happen is you go, don't care, don't care, don't get don't care up to some threshold: care massively. And that's it, right? And what spectral risk measures do is they give you the ability to be much more nuanced about that. And they say No, I care about all the losses, they care about even the ones that are better than that, I just don't care about them so much. So rather than having a caring function that goes don't care, you know, care a lot, it's a gradually increasing thing and spectral risk measures give you a consistent, broad set of families to do that, that allow you to express much more nuanced risk appetites than all or nothing.
David Wright:Okay, so that reminds me actually, about something that I promised already into myself. We were touched on, which is Delbean, Delbaen. I cannot pronounce his name, Freddie. Our boy, Freddie. So he wrote this tremendous paper, tremendous, in all sorts of ways. Unbelievably dense paper that I don't understand. And, you know, even, you know, even took a man as great as Steve Mildenhall 20 years to figure it out. But like, So, explain it to me, Steve. Like he figured something out a long time ago that took the industry a heck of a long time to digest and understand the consequences of. What is it that he understood?
Steve Mildenhall:So he was one of the co authors in a paper that I think came out in 1997, called Thinking Coherently. And it introduced the axioms for coherent risk measures. And the idea was they said, Okay, we're trying to measure risk. There should be certain things we can agree on about risk. And let's write those down as axioms and they were basically: a pool is less risky than the sum of the parts. Seems reasonable, right? You got you got some diversification going on. If a certain risk is less than a certain other risk in every state of the world then the risk measure should be less, right. It's called monotonic. If you add a constant to a risk, you're just like translating it, there's nothing else going on there. And if you scale a risk, the risk scales, right. And that one is a bit dubious. But you know, let's stop that. So you've got these four axioms. And they define what's called a coherent risk measure. And what Delbaen's paper then does is it says, Alright, these are exactly all of the coherent measures, right? It characterizes them and the way it characterizes them, it says they're all characterized as the worst risk adjusted expected outcome, where the risk adjustment, which is a way of adjusting your objective probabilities, lives in a certain convex set. Okay, so that is your characterization of all coherent measures. And basically, everything follows from that. And as John said, the sort of fundamental building block is, they're all TVaRs anyway, so it's all about different weighting functions on TVaRs. And it's a matter of how you come up with the weights.
David Wright:And there's what there's one kind of part of the paper where he talks about whether some scenarios exist. And if something exists, then the marginal contribution of a risk to the whole is calculable. Am I understanding that right? That? If I even phrased that question, right?
Steve Mildenhall:I'm not sure from that description.
David Wright:So it's a Q with like a shadow on it is I'm gonna be very specific and embarrass myself.
Steve Mildenhall:P's and Q's. P is your objective probability, Q is your risk adjusted probability.
David Wright:Okay. And I think it's like Q of Y or something, or negative Q of y. And if this if this exists, and there's ways multiple ways for it to exist,
Steve Mildenhall:yeah. Okay. That's, that's, that's it. That's it. That's it. Okay. So what that's about is, is it saying, for any, so you got your spectral risk measure. You... it is a risk adjusted expected value, right? And the risk adjustment is your Q is this set of adjusted probabilities. The key result is, if there's only one way of coming up with those risk adjustments, then everything works. And in particular, the risk adjusted probability method, and the marginal method goes all the way back to you know, Myers Read marginal method, everyone's likes marginal methods, they are the same. But if there are multiple ways of doing the risk adjustment, then they are different. And what's going on there is basically you've got a flat spot in your outcome. So if you think about TVaR, let's say you're looking at 90 99% TVaR, if your outcome distribution, which takes the same value, say, between the 98 and a half, and the 99th and a half percentile, so you got a little flat spot, then it's not obvious what the worst 1% of outcomes is, right?[Okay], you've got a choice, you've got a 1% band, and you've got to pick half a percent of it. And you can do that in a lot of different ways. Now, just because your total is constant, doesn't mean that each of the lines, the units that go into that total are going to be constant. So it really matters which half a percent you pick, right? And that is the reason why the allocation is not unique. And depending on which part you pick you have different allocations, it accounts for all the order uncertain, you know, ordering problem, accounts for if you grow or shrink, you get different answers and everything. And it basically boils down to the fact that there's no unique derivative, you've got a function that looks like this. And if you approach it from this way, you think the slopes here, and if you approach it this way, you think the slopes here, and you've got a whole range of slopes that correspond to picking different half of the same bands out of that 1% flat spot. And that that was really only appreciated, like in the early 2000s, after you know Delbaen had the paper, but the characterization of it essentially goes back to no flat spots was a couple of other papers that were in the early 2000s.
John Major:And there's quite a bit of ink spilled in the book detailing this phenomenon.
Steve Mildenhall:Yes, this is hopefully some diagrams that make this clear and what have you. Yeah,
David Wright:so So I get the problem. What's the solution, the resolution to the problem? So like we approach it for different sides of slope looks different. How do you handle that?
Steve Mildenhall:So we offer two solutions to that problem. One is, the reason you've got a flat spot is generally because you've got something like you're you've got a default situation. So you can only pay the losses. You know, you can only pay up to the assets you have or maybe you've got like an an aggregate stop. You've got a layer of reinsurance, [okay] it causes your outcomes to always be constant, but in It is often the case that you can look through that you can say, ah, actually, there's this sort of unlimited random variable sitting out there that has no flat spots. And that determines a unique ordering on all events, because the key thing here is to rank all of your outcomes from worst to best. That's the accounting that you've always got to do. And when you've got ties, you've got a problem, right? How do I order within the ties that that is what drives the problem. So if you can go, if you can lift it, we call it the lifted allocation, if you can go back to a sort of bigger it like a gross risk that doesn't have a flat spot, you use that, and you get rid of the problem. So that's one solution. The other solution is essentially that you just average all the ways you can permute in the background. And that's just linear natural allocation. Those are the two solutions that we have.
David Wright:So what I like about that. But the first one. Second one, not sure I like but the first one is because you're using real insurance knowledge, you're looking at that you're saying something's going on here that's real. This is not just a mathematical weird anomaly. It's not like, you know, I don't know, it's it's not some strange function that behaves Strange is not enough, because we put an aggregate stop loss on that thing. So there's like a real trigger for this, we gotta go back to the assumptions or not the assumptions, but I don't know the the components of the model, and then we can get some insight from there. That's great. [When it's available, you can do that.]
John Major:[Yeah, yeah] it's not always available.
Steve Mildenhall:The bummer here is, is that if you are a Limited Liability Insurance Company, which all insurance companies are right, they have a finite amount of assets, you always have a flat spot, if you have the possibility of default, which you basically always do. So essentially, the marginal method never, never really works. You have to do something to get around it.
David Wright:Okay, so guys, let's talk about the book. So the the so here it is. I have a copy too, of course, which I was very pleased to get in the mail because I got pdf copy. This is way better. How long did it take? How did you guys start it? What on earth would possess you to write a? Let's do a quick check here. 500 Something page book of math.
John Major:It all started with Don Mango.
David Wright:Okay.
John Major:I was I was, as I mentioned before, I was working with him on refining the capital tranching concepts. And, you know, we got to the point where he thought this would this would be a great thing to show at a CAS conference. And he roped Steve in, he thought, you know, there's another guy who would be really good for this. Let's bring Steve Milton Holland. And so we did and what was it like from from your end, Steve? I had actually met Steve years earlier in an NBER meeting, but since we were both working for competitors, it was kind of an arm's length Oh hi, how you doing?
Steve Mildenhall:Yeah. Whereas until 2016, I worked for Aon, Aon Benfield, and John was working for a Guy Carp. So I used to keep a very careful eye on exactly what they did. And and basically look for holes in it. It was a competitive issue. So I remember John being fairly frosty at that meeting. And but by 2016, I moved to St. John's. So I was now neutral territory. And yeah, I remember Don, giving me a call saying that they had a spot on this team that wanted to do this. And I had been already sort of toying around I, I have a manuscript, I think that goes back about 2012 for a monograph that was called All About Capital that was basically a precursor to this book. I've been thinking about, you know, wanting to write this stuff up. And when I moved to academia, one of the things I was keen to do was, you know, let me try and find the answer that I'm sure exists in the literature and, and spend the time on it. So it was great to Yeah, it was an incredibly productive collaboration with John. We use GitHub to share files back and forth. I just looked up and the book took 1000.
John Major:Well, let's talk about the meeting. Let's talk about the meeting. And this This was a multiple day session. It wasn't just an hour, this was multiple hours presenting at CAS, it was a deep dive, it was called The Most Important Risk Measures You Never Heard Of. And it was, you know, our intro introducing spectral risk measures to the audience and the videos that you alluded to, were made of that, of that couple of days there. And we came out of that and went wow, that went pretty well. And, you know, maybe we should write this up. And the first idea was maybe a monograph, you know, maybe maybe a CAS monograph about 100 pages, that ought to do it. And but Steve had bigger ideas.
David Wright:What was the bigger idea? Like why, why, why
John Major:everything you want to know about capital and risk.
Steve Mildenhall:I wanted the stuff at the beginning about risk measures because that's not well covered in the syllabus. I wanted the history that we've talked about a little bit, you know, the Wang papers, the Phillips the Myers-Cohn, Myers-Read all that stuff to give the context. And there's just a lot of stuff here when you when you're going to go through the details, you know, we've we've structured it around, it's basically three parts. Part one is risk measures. Part two is pricing a portfolio, coming up with that total cost. And then part three is allocation, there is a part four of miscellaneous other topics. And then within each part, we divided into what we call the classical period, which is up until 97, which was your Wang's layering paper, the Phillips Cummins and Allen paper on multi line pricing, and the coherent risk theory paper. So that's a nice dividing line. So we do the classical - the way we used to think about it, theory, and then how that worked in practice. And then we do the new, the modern approach, both in theory and practice. So there's sort of four subsections involved, one for portfolio and one for allocation.
David Wright:This is right, I think, Steve, you've bragged to me about how this is all contained in like a IPython notebook or something like that, where you can generate all the graphs, a humongous number of graphs. And you know, all these examples, right? So this is all stuff you've had to invent.
Steve Mildenhall:[Right?] Yeah, I have a package called Aggregate that I used to generate all the examples in the book. And frankly, I actually used it more to, to understand the theory, I spent countless hours, just computing things and looking at them and trying to understand what's going on. I mean, the question about... alright, so you take a cat line and a non cat line, and you pool them together, and most of the diversification benefit goes to the non cat line. Well, why? Why does that happen? I've spent a lot of time thinking about that. I think we've got to a good place in the book sort of to answer that. And you know, the answer is, yeah, it does. Partly because the cat drives the tail. And the tail is expensive, as we've discussed. But the degree to it actually varies with with your risk tolerance and your risk appetite, and you can charge more or less on the non cat line. So yeah, creating the software was a big part of that. I'm currently working on writing that up. And I hope to release that before the end of the year, and maybe get people use it. So they will, for example, be able to reproduce a complete set of the exhibits. For the we've got four case studies, we run through the book, each with two lines, it's always a less volatile and a more volatile line. So fairly simple, but a lot of fairly sophisticated exhibits. And you'll be able to reproduce all of those for the book case studies. And for your own case studies using the software once it gets released.
David Wright:And I, I love I worry a little bit about something with this book, which is that this inevitably, some chunk of his maybe tons of chunks of it are going to wind up on the CAS syllabus. And that's going to be great for for the future of humanity. Let's call it so it's good. But I wonder whether the history is going to do because of the history is great. I mean, you guys have I think we don't have enough of a sense of history in this business, where we don't think hard enough about the alternate universes that already existed in the previous decades, when you have different kinds of regulatory regimes, things that could happen again, in some way or another. Right? So to learn the history, I think is such an important thing. And I don't think we do quite enough of that, except on one of the exams in the actuarial syllabus where we talk about the history of regulation, which was my favorite part of the whole thing. It used to be exam six anyway when I took it. And so I love the history, I hope, you know, people continue to study it. And and also kind of understand the we can honor the names of the people who did who discovered this stuff. We don't have enough, I think enough of a pantheon either in our business. And maybe you can take a moment to sort of talk a little bit about that. And who are the who are the people who would thank him and some of them have passed on and we'll talk about Don, but are there other people that you know, we want to celebrate? contributed to this?
John Major:You wanted to mention Glenn didn't you?
Steve Mildenhall:Yeah. Oh, yeah. Well, I was just wondering if you wanted to go first. But I'm happy to jump in. So I mean, the history part of it to me is this has been my career like this has been my, my professional life has been figuring this stuff out. I've been thinking about risk, basically, since day one. You know, very early on I was worked on the allocation of charges across state and line when I was in personal lines at CNA. We've mentioned quite a few people, you know, Shaun Wang, obviously hugely important, the people I interacted with at the Risk Theory Seminar. So David Cummins was there, Jim Garvin, Steve D'Arcy, and then Richard Derrig I've mentioned a few times from from the AIB. The one thing that hasn't come up so far that was just really, really important for me is Glenn Meyers, who strangely didn't work for a reinsurance broker, is outside that loop. But I, through talking with Glenn, and through reading his papers, sort of informed how I think about modeling insurance risk and how you factor the uncertainty components into it, how you think, economically, your marginal cost and marginal revenue kind of ideas. So he was just just hugely influential in this. And it was through those discussions that was immediately apparent to me when I read the Myers Read paper, oh, they've made this homogeneous assumption. Well, that's not how it works, right? You don't grow by scaling up, you grow by adding new, independent or largely independent insureds to your portfolio. So the distribution changes shape. Glenn Meyers would never have made that assumption, because it's just simply not how insurance works. And if you've been trained to think the way he thought it was just obviously not the right way to do it.
David Wright:I can I just make one point. And then John, I want to get your reaction to the same kind of topic. But the the the concept of you having personal relationships with people and conversations with people, right? as informing your intuition I think is easily overlooked. Actuaries, we can talk about sort of the culture of the business. You know, one of the things I noticed that's different about actuaries early on in their exam taking process and the people who make it out the other end, is the ones who make it out the other end are much... if I could just put it this way: more fun to hang out with. Or they you know, they're just there's a sociability, I think about succeeding in anything in life. And including being an actuary, think of it as a mathematical profession, and is that of course, but you know, the intuition that you develop that allows you to really make real contributions and actually see straight through a very high status pair of people putting a paper out, to flaws in their assumptions based on the conversations you had with a mentor of yours. I want to emphasize that. How did you meet Glenn? How do you how did you come to have these conversations? I didn't have conversations like this early in my career. What did you do that I did wrong? That I didn't do
Steve Mildenhall:I shudder to think how obnoxious I was at the time, but I heard Glenn telling this story to someone else. And I'm not sure if he knew I was I was within earshot. But he said, you know, he was I was at the Boston CAS meeting I think in 95, when I got my fellowship, and I read his stuff, and I knew who he was. And I was like, I had a question for him. And I just went up to him, and I asked him. I can't even remember what it was. So you say, Oh, well, what about this? And you know, and he was great. I think, you know, I think all researchers love it when people read their material. And he is all about the problem solving. And he just doesn't.. Oh, let me you know, in his Glenn way, let me explain to you how that all works. And it was fantastic. I just learned so much from him. He was great.
David Wright:John, how about you?
John Major:Oh, I was fortunate enough to be working alongside and for some some real superstars there. Gary Venter, Rodney Kreps. And then they would in turn would go and bring in people as consultants like Shaun Wang and Ken Froot and I get to interact with them. So I just lucky enough to be in the right place at the right time to interact with these people.
David Wright:And so many, so many things are, I guess. So let's kind of close on this sort of concept of what are some what can people do to help the next kind of level of this cause, you know, like, what are some what are some interesting problems that are outstanding? What What advice would you give, let's say, you know, the earlier version of yourself, you know, what, where should they go? What's the next level of development? What would you do if you were 24 years old? 25 years old and looking for cool things to work on? Because it ain't done? Right?
John Major:Okay, yeah. This may not be exactly what you were looking for. But I think at this point we've kind of pulled the curtain back on this new way of looking at things and it needs we need people to start playing with it and using it and kicking the tires and seeing how you can apply it profitably and and what kind of problems you can get into if you're, if you're abusing it. I don't want to try and talk about what theoretical directions new development needs to be done I think I think it's implementation is the is the next question now let's let's have a revolution and overturn the the way things have been done. Let's Let's sweep away this notion of allocating capital and applying a constant cost of capital rate to it and let's let's start thinking this new way and seeing if we can actually make it work.
Steve Mildenhall:Yeah, I guess I'd add that risk is subjective. And the theory is trying to model the reality that emerges in the market. And this actually reminds me of, I remember talking with with Richard Derrig about one of the big company in Massachusetts, who, you know, they have a dominant market share. And I'm like, Man, I wish I was inside there, they definitely know what they're doing. And Richard just looked at me and said, What makes you think they know what they're doing? They're just as in the dark as anyone else. So you, actually tend to be sort of strong Bayesians. And they often go in with these sort of theoretical prejudices. And I think we've got to remember that the theory follows the practice, it's trying to model a reality that exists outside the theory. And you learn so much from that by listening to what people say, and watching what they do, and particularly senior people, right, because Joe Actuary isn't going to change the direction of the company. But if a CEO of a big insurance company decides or the senior management decide they don't like cat risk, that's gonna have a market impact, who's going to withdraw capital from it, the prices are gonna go up. So what they think and how they describe risk and what have you, you need to make sure that you've got a model of risks that can incorporate the types of things that they're saying, and don't go and think oh, you know, I'm I've done the exam on this, I know how this works. And that doesn't make any sense. Well, doesn't matter whether it makes sense or not, it's going to drive the market. If you're trying to model the market, you need to be able to incorporate it. So listening to what... your market participants say about risk with an open mind and thinking about how to incorporate it into theory, I think is a key to success in the future.
David Wright:Brilliant, and having a sense of history, which you can get from among other things, Pricing Insurance Risk and also other work you guys have collaborated on, which we've done podcasts on Steve of the history of the insurance marketplace, and that's often a real good way to to communicate with folks about you know, how does this fit into what happened? We'll end it there, guys. My guests today, Steve Mildenhall, John Major for the marathon Not Unreasonable, I think probably coming in at two and a half hours or so, their book, Pricing Insurance Risk. I loved it. Very proud to have done this with you, fellas. Thank you very much.
Steve Mildenhall:Thank you